Cybernetic Learning Systems Group
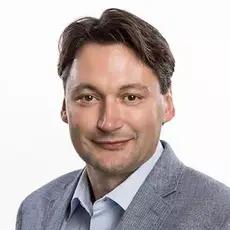
“Cybernetics is the study of interaction and feedback processes, which drives our approach to developing AI solutions for complex systems. We develop AI agents which can interact with their environment, communicate and cooperate with other agents, and learn from feedback. Combining human expertise and creativity with the processing power of AI allows us to create new solutions for the problems of today and tomorrow.”
Fields of Expertise
- ML for autonomous systems & robots
- Methodical focus on RL
- Reinforcement Learning
- AI-supported decision making
- Intelligent transportation systems and aviation
- Human-AI interaction and co-learning
- Embodied AI
- Multi-agent systems
Our group boasts a wide range of expertise, given the diverse backgrounds of our team members. We develop AI systems which have a positive influence on society and benefit humans, assist in complex environments, automate processes and push the frontier towards collective intelligence. Our focus lies on the development of AI approaches inspired by biological and feedback learning, hence the name “cybernetics”. David A. Mindell defined cybernetics as “the study of human/machine interaction”, whereby he states the core idea that most any system can be analyzed using the principles of feedback, communication and control, which we emulate in our research. Developing holistic approaches to intricate problems requires the usage of more than one tool, which is why we leverage the best suited approaches, ranging from multi-agent, distributed and hierarchical to causal RL. Our AI-agents should sense and interact with both their environment and other human and artificial agents within it, while behaving in a predictable and reliable manner. Our fields of applicability are virtually unlimited; integrating AI into transport systems such as aviation and rail, supporting human decision-makers, fully automating processes and robotics, to name a few. It is essential to us to maintain human agency and control over systems, ensuring that human domain knowledge and expertise is not lost or replaced, but supported and enhanced.
Also see: https://cyberneticlearningsystems.github.io/
Services
- Insight: keynotes, trainings, public outreach, scientific diplomacy
- AI consultancy: workshops, expert support, advise, technology and ethical assessment
- Research and development: small to large-scale collaborative projects, third party-funded research, student projects, commercially applicable prototypes
Team
Projects
As part of the reorganization of the research database, the previous lists of research projects are no longer available. Die Zukunft geht in Richtung Volltextsuche und Filterung, um bestmögliche Suchergebnisse für unsere Besucher:innen zur Verfügung zu stellen.
In the meantime, you can easily find the projects via text search using the following link: «To the new search in the project database»
Publications
- Previous Page
- Page 01
- Page 02
- Page 03
- Page 04
- Next Page
-
2007.
Bern:
UniPress.
-
Timmermann, Axel; Okumura, Y.; An, S.-I.; Clement, A.; Dong, B.; Guilyardi, E.; Hu, A.; Jungclaus, J. H.; Renold, Manuel; Stocker, T. F.; Stouffer, R. J.; Sutton, R.; Xie, S.-P.; Yin, J.,
2007.
The influence of a weakening of the Atlantic meridional overturning circulation on ENSO.
Journal of Climate.
20, pp. 4899-4919.
Available from: https://doi.org/10.1175/JCLI4283.1
-
Mölg, Thomas; Renold, Manuel; Vuille, Mathias; Cullen, Nicolas J.; Stocker, Thomas F.; Kaser, Georg,
2006.
Geophysical Research Letters.
33(18).
Available from: https://doi.org/10.1029/2006GL026384
-
Yoshimori, Masakazu; Raible, Christoph; Stocker, Thomas; Renold, Manuel,
2006.
Climate Dynamics.
27(5), pp. 493-513.
Available from: https://doi.org/10.1007/s00382-006-0144-6
-
Yoshimori, Masakzu; Stocker, Thomas; Raible, Christoph; Renold, Manuel,
2005.
Externally forced and internal variability in ensemble climate simulations of the Maunder Minimum.
Journal of Climate.
18, pp. 4253-4270.
Available from: https://doi.org/10.1175/JCLI3537.1