Research by CAI member published at NeurIPS
The paper “Safe Time-Varying Optimization” based on Gaussian Processes with Spatio-Temporal Kernel will be presented as poster during NeurIPS 2024 in Vancouver.
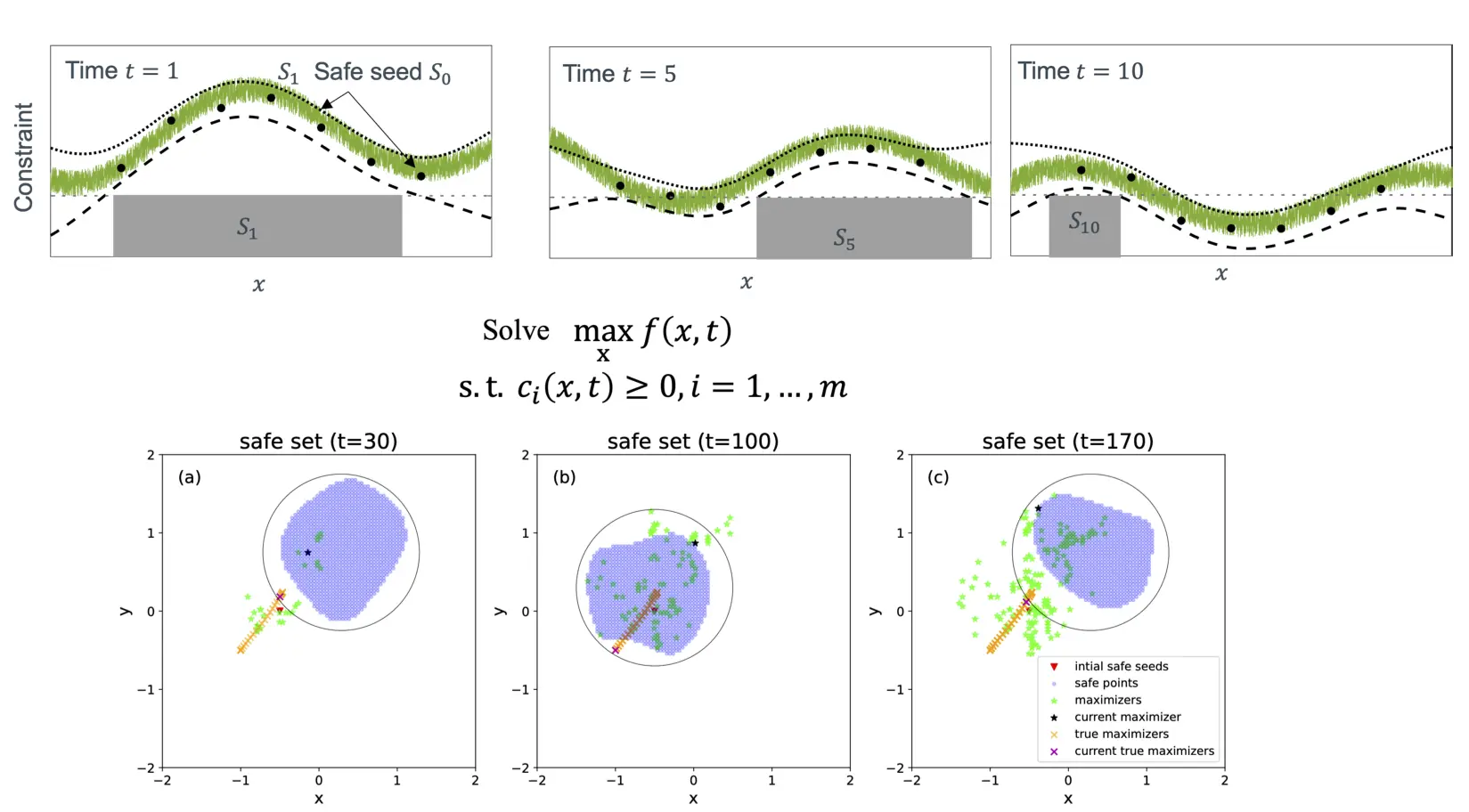
Ensuring safety is a crucial in sequential decision making problems, such as medical dosage design, online operation of chemical plants, autonomous racing, or robotics contol in general. The complexity of the underlying systems often makes finding the optimal decision challenging, especially when the safety-critical system is time-varying. The proposed algorithm, called TVSAFEOPT, overcomes the problem of optimizing an unknown time-varying reward subject to unknown time-varying safety constraints. The algorithm is based on Bayesian optimization with a spatio-temporal kernel, and is capable of safely tracking a time-varying safe set without the need for explicit change detection. Optimality guarantees are also provided for the algorithm when the optimization problem becomes stationary. Evaluation on a realistic case study with gas compressors confirms that TVSAFEOPT ensures safety when solving time-varying optimization problems with unknown reward and safety functions.
The Conference on Neural Information Processing Systems (NeurIPS) is a machine learning and computational neuroscience conference held every December. Along with ICLR and ICML, it is one of the three primary conferences of high impact in machine learning and AI research.
This work is a collaboration of the IAI group led by Alisa Rupenyan at CAI, with researchers at ETH Zurich and TU Delft.
For more details, check the paper here, and the poster here.