Transforming clinical assessments: Explicitly articulating implicit clinical decision-making to train AI
We are developing an AI algorithm to assess movement quality after strokes based on video recordings. To achieve this, we evaluate the reliability of 25 therapists who rate compensatory movements in videos. The goal is to establish a reliable foundation for AI-supported clinical decision-making.
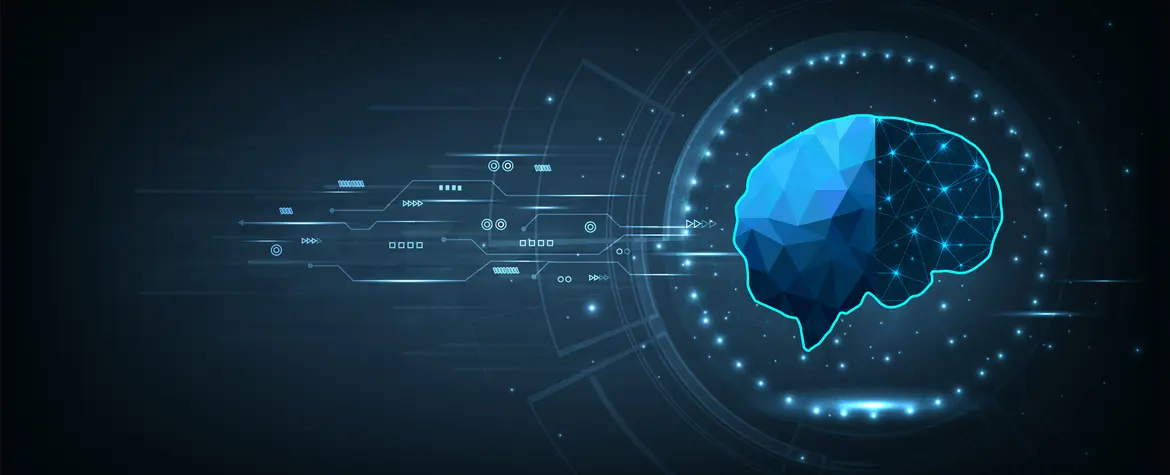
Description
We are currently creating an AI algorithm using modern computer vision methods to assess movement quality in people after stroke. Our project, as many others, depends on therapist’s manual movement quality rating to create the ground truth. While therapists are trained to reliably assess movement quality in person (3-dimensional, 3D), it is unclear if they can do so when rating is based on videos (2-dimensional, 2D). The aim of this nested DFF project is to assess the reliability of video-based observations when evaluating compensatory movements in the upper extremities and trunk during a drinking task performed by post-stroke clients. Therefore, we recruit 25 therapists to assess 7 anonymized video recordings of patients after stroke and let them rate compensatory movements on a scale. We will analyze intra-rater and inter-rater reliability to contribute to reliable ground truth in AI applications for clinical decision-making processes.
Key Data
Projectlead
Deputy Projectlead
Project team
Celina Chavez (Donau Universität Krems), Dr. Elena Gavagnin, Benjamin Kühnis
Project partners
Donau Universität Krems; Lake Lucerne Institute AG
Project status
completed, 02/2024 - 12/2024
Funding partner
ZHAW digital / Digital Futures Fund
Project budget
18'000 CHF
Further documents and links
Publications
-
Evaluating inter- and intra-rater reliability in assessing upper limb compensatory movements post-stroke : creating a ground truth through video analysis?
2024 Sauerzopf, Lena; Chavez Panduro, Celina G.; Luft, Andreas; Kühnis, Benjamin; Gavagnin, Elena; Unger, Tim; Easthope Awai, Christopher; Schönhammer, Josef G.; Degenfellner, Jürgen; Spiess, Martina
-
Transforming clinical assessments : rater reliability in video-based evaluation of upper extremity after stroke
2024 Sauerzopf, Lena; Chavez Panduro, Celina G.; Luft, Andreas R.; Kühnis, Benjamin; Gavagnin, Elena; Unger, Tim; Easthope Awai, Chris; Schönhammer, Josef G.; Degenfellner, Jürgen; Spiess, Martina
-
Movement analysis through videos in occupational therapy : insights from an ongoing research project for clinical practice
2024 Chavez Panduro, Celina Gabriela; Spiess, Martina; Gavagnin, Elena; Kühnis, Benjamin; Unger, Tim; Schönhammer, Josef; Sauerzopf, Lena