Automated Grading with AI?
In their Bachelor’s thesis, ZHAW graduates from the ZHAW Data Science and Informatics programs analysed the automatic creation of open examination questions and their evaluation. They investigated various automatic grading systems and large language models (LLMs) and present their work currently at the renowned Computer Science Education Conference (SIGCSE 2024) in the US. How do AI systems perform compared to humans?
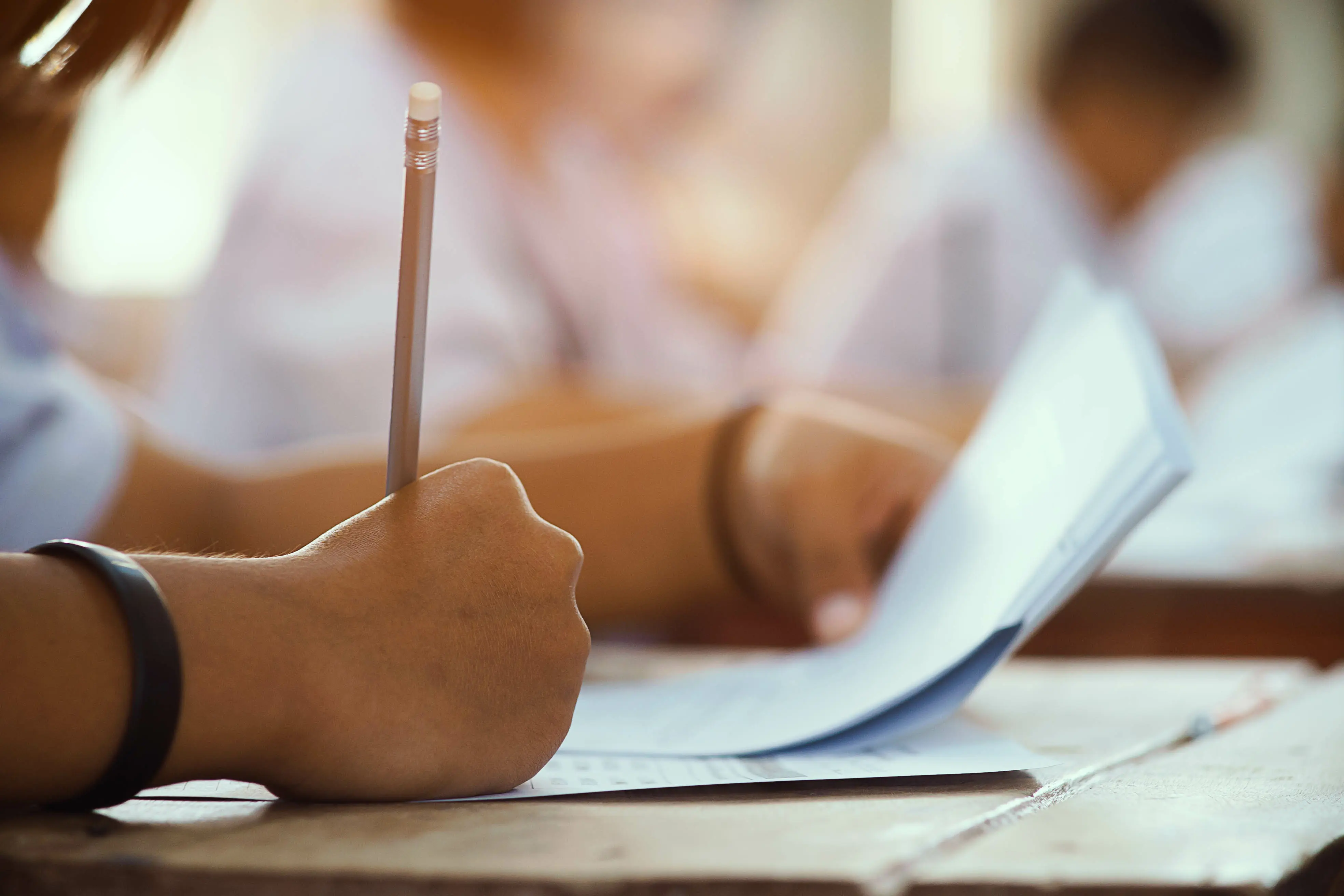
Open-ended questions promote a deeper understanding in written exams than multiple-choice questions and are often the preferred assessment method. However, their evaluation is more time-consuming and less objective than, for example, grading multiple-choice answers. This is why there are efforts to automate the grading process using so-called Short Answer Grading (SAG) systems. To assess the capabilities of these systems, ZHAW students Gérôme Meyer and Philip Breuer, under the supervision of Dr. Jonathan Fürst, developed a new benchmark.
Human vs. Machine: Evaluating Assignments
The paper was accepted at the prestigious SIGCSE conference, which is supported amongst others by Google, Microsoft, and the U.S. National Science Foundation, and focuses on topics related to computing education. On December 5, the Data Science students present their preliminary work, which aims to facilitate the comparison of automatic grading systems. In it, they combined seven commonly used English short-answer grading datasets, which included a total of 19,000 datasets with questions, answers, and grades. This allowed them to evaluate a range of current SAG methods. The results showed that AI-based approaches, while promising, are still a long way from human performance. The insights gained by analyising these results on more detail open up new avenues for future research on human-machine SAG systems.
Future Questions
Other findings from the study are that Large Language Models (LLMs) are transferable to different situations and can evaluate new questions. Specialised grading systems have more difficulty doing this – in these cases, the AI needs to be "fine-tuned", i.e., specifically trained. Moreover, the ability to generalise improves as the size of an LLM increases. In a next step, the students plan to address the problems they encountered during their work. They want to extend the benchmark in the future with more diverse datasets to reduce biases, include multilingualism, and conduct a more thorough evaluation of automatic grading solutions.
So it will be some time before university exams can be created and marked fully automatically. Ultimately, work like this gives us solid insights into the possibilities and limitations of artificial intelligence in concrete applications - how we use it is up to us humans.
More Information
- Conference: ACM Virtual Global Computing Education Conference (SIGCSE VIRTUAL)
- Preprint: ASAG2024: A Combined Benchmark for Short Answer Grading
- Benchmark from the work: ASAG2024