Mohammadreza Amirian successfully defends PhD thesis on trustworthy AI
The dissertation on “Deep Learning for Robust and Explainable Models in Computer Vision” brought about important discoveries over a series of applied research projects with practice partners and was evaluated with “magna cum laude” at Ulm University, Germany.
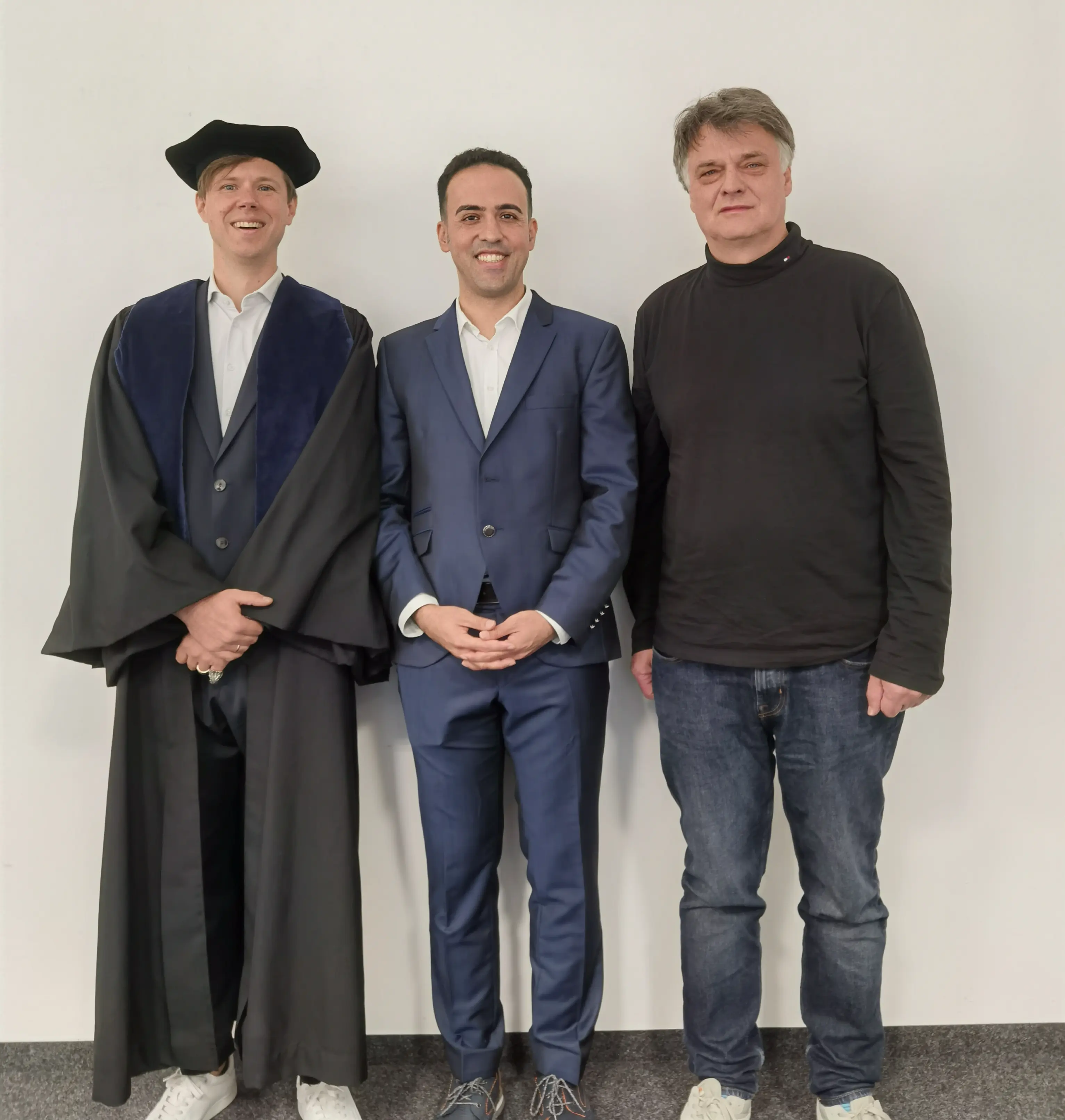
On October 20, 2023, Mohammadreza Amirian, who worked at InIT/CAI between 2017 and 2023, successfully defended his PhD thesis on "Deep Learning for Robust and Explainable Models in Computer Vision" at Ulm University with an excellent talk & disputation, leading to the evaluation “magna cum laude”. The Phd committee comprised the co-supervisors Prof. Thilo Stadelmann and Prof. Friedhelm Schwenker, Profs. Guenther Palm, Daniel Braun and Hans Kestler of Ulm University, and Prof. Martin Jaggi of EPFL as external reviewer. The committee was headed by Prof. Matthias Tichy.
He accrued an impressive track record over the time of his PhD studies, including 7 peer reviewed journal publications and 8 peer reviewed conference papers, 6 of those publications as first author. Amongst other things, he worked on affective computing (in conjunction with Ulm University’s Institute for Neural Information Processing), was a driving force in a team that competed (and scored amongst the top5) at Google’s AutoDL challenge 2019, and improved machine-learning-based motion artifact compensation for computed tomography images as the main collaborator. He also contributed to the theory and implementation of deep radial basis function networks, rotation-invariant vision transformers, and uncovered root causes behind bias in face recognition systems (to name a few of his projects).
Mohammadreza’s thesis presents developments in computer vision models' robustness and explainability. Furthermore, it offers an example of using vision models' feature response visualization (models' interpretations) to improve robustness despite interpretability and robustness being seemingly unrelated in the related research. Besides methodological developments for robust and explainable vision models, a key message of his thesis is introducing model interpretation techniques as a tool for understanding vision models and improving their design and robustness. In addition to the theoretical developments, the thesis demonstrates several applications of machine and deep learning in different contexts, such as medical imaging and a affective computing.
On behalf of the CAI, we extend our heartfelt congratulations to Mohammadreza Amirian.