CAI takes a look at AI‘s role in the energy transition
AI can help to better infer sub-surface conditions for safe use of geothermics; of course it should become more energy-efficient itself.
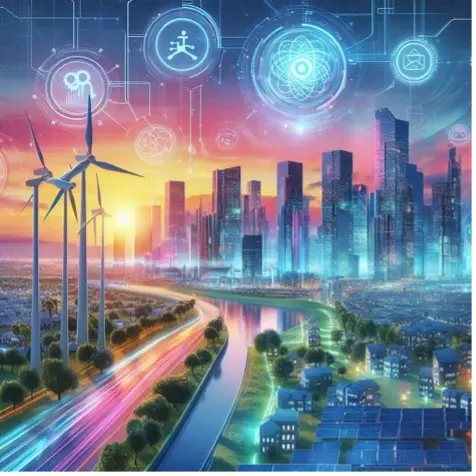
In a recent collaboration between the ZHAW Centre for Artificial Intelligence (CAI) and Karlsruher Institut für Technologie (KIT), we analyzed different machine learning algorithms to help with predictions of sub-surface conditions relevant for the exploitation of geothermics. Besides, the ML-based inference reduced the amount of compute necessary by six orders of magnitude compared to traditional simulation models.
Here's the abstract from the paper recently published in Elsevier's Geothermics journal:
Including uncertainty is essential for accurate decision-making in underground applications. We propose a novel approach to consider structural uncertainty in two enhanced geothermal systems (EGSs) using machine learning (ML) models. The results of numerical simulations show that a small change in the structural model can cause a significant variation in the tracer breakthrough curves (BTCs). To develop a more robust method for including structural uncertainty, we train three different ML models: decision tree regression (DTR), random forest regression (RFR), and gradient boosting regression (GBR). DTR and RFR predict the entire BTC at once, but they are susceptible to overfitting and underfitting. In contrast, GBR predicts each time step of the BTC as a separate target variable, considering the possible correlation between consecutive time steps. This approach is implemented using a chain of regression models. The chain model achieves an acceptable increase in RMSE from train to test data, confirming its ability to capture both the general trend and small-scale heterogeneities of the BTCs. Additionally, using the ML model instead of the numerical solver reduces the computational time by six orders of magnitude. This time efficiency allows us to calculate BTCs for 2′000 different reservoir models, enabling a more comprehensive structural uncertainty quantification for EGS cases. The chain model is particularly promising, as it is robust to overfitting and underfitting and can generate BTCs for a large number of structural models efficiently.