Rieter Endowed Professorship for Industrial Artificial Intelligence
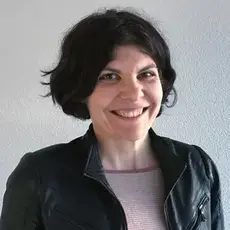
"We focus on developing learning-based control and optimization methods that integrate expert knowledge and symbolic relationships to optimize industrial manufacturing processes, enhance autonomy, and ensure safety in the face of complex dynamics and variability."
Dr. Alisa Rupenyan, Rieter Endowed Professorship for Industrial Artificial Intelligence
The Rieter endowed professorship at the Centre for Artificial Intelligence has a particular focus on the development and application of principled, neuro-symbolically inspired learning in conjunction with processes in production and service. This combines machine learning with knowledge-based systems.
Fields of Expertise
- Learning-based control and optimization of manufacturing systems
- Neuro-symbolic AI for modeling and optimization of manufacturing processes
- Expert knowledge integration in machine learning models
Industrial manufacturing processes pose various challenges to their optimization and control, due to their complex dynamics, lack of observability, and large variability. Apart of finding the right optimization approaches and parameters, finding the right strategy to adapt to different requirements, different components of the equipment, or changes in the environment is crucial. Leveraging existing information in the form of graph-based contextual information, logical rules, or another abstracted form of pre-existing knowledge is very promising to alleviate the large variability challenge. The research focus of the Industrial AI (IAI) group is on the development of learning-based control and optimization methodologies, incorporating the existing expert knowledge, and symbolic relationships in the process, tapping into the newly emerging neuro-symbolic AI approaches. Our goal is to demonstrate that industrial processes can be optimized, combining machine learning models (such as neural networks) and symbolic reasoning (graph-based representations, logical rules), and methods from control and sequential decision making, as well as domain expertise. We are interested in achieving increased autonomy for systems in various domains (manufacturing, construction, process industries), and working with distributed systems, while striking a balance between autonomy and human oversight. By developing innovative solutions that leverage both quantitative data and qualitative domain understanding, we contribute to more adaptive, efficient, and safe industrial processes across various domains.
Services
- Insight: keynotes, trainings
- AI consultancy: workshops, expert support, advise, technology assessment
- Research and development: small to large-scale collaborative projects, third party-funded research, student projects, commercially applicable prototypes
Team
Head of Research Group
Projects
Unfortunately, no list of projects can be displayed here at the moment. Until the list is available again, the project search on the ZHAW homepage can be used.
Publications
-
2024.
KI-Trick zur Bereinigung von Maschinendaten.
Aktuelle Technik.
Available from: https://www.aktuelle-technik.ch/ki-trick-zur-bereinigung-von-maschinendaten-a-201dd57454851223b1f74e9f5c047dae/?cmp=beleg-mail&pt=673c351e81d26
-
Li, Jialin; Zagorowska, Marta; De Pasquale, Giulia; Rupenyan-Vasileva, Alisa; Lygeros, John,
2024.
Safe time-varying optimization based on Gaussian processes with spatio-temporal kernel [paper].
In:
Conference on Neural Information Processing Systems, NeurIPS 2024, Vancouver, Canada, 10-15 December 2024.
Vancouver:
NeurIPS.
Available from: https://doi.org/10.21256/zhaw-31795
-
Yan, Jiaqi; Chakrabarty, Ankush; Rupenyan, Alisa; Lygeros, John,
2024.
In:
IEEE 20th International Conference on Automation Science and Engineering (CASE).
IEEE 20th International Conference on Automation Science and Engineering (CASE), Bari, Italy, 28 August - 1 September 2024.
IEEE.
pp. 1910-1915.
Available from: https://doi.org/10.1109/CASE59546.2024.10711717
-
Liao-McPherson, Dominic; Balta, Efe C.; Afrasiabi, Mohamadreza; Rupenyan-Vasileva, Alisa; Bambach, Markus; Lygeros, John,
2024.
Layer-to-layer melt pool control in laser powder bed fusion.
IEEE Transactions on Control Systems Technology.
Available from: https://doi.org/10.1109/TCST.2024.3464118
-
Nobar, Mahdi; Keller, Jürg; Rupenyan, Alisa; Khosravi, Mohammad; Lygeros, John,
2024.
Guided Bayesian optimization : data-efficient controller tuning with digital twin.
IEEE Transactions on Automation Science and Engineering.
Available from: https://doi.org/10.1109/TASE.2024.3454176