5th European COST Conference on Artificial Intelligence in Finance and Industry
We would like to welcome you to the «5th European COST Conference on Artificial Intelligence in Finance and Industry», hosted by the Institute of Applied Mathematics and Physics (IAMP) and the Institute of Data Analysis and Process Design (IDP) at the School of Engineering, and the Institute of Wealth & Asset Management (IWA) at the School of Management and Law of the Zurich University of Applied Sciences (ZHAW) in Winterthur, Switzerland.
Artificial Intelligence in Industry and Finance (5th European Conference on Mathematics for Industry in Switzerland)
September 3, 2020, 12:30-17:30 - Online Conference
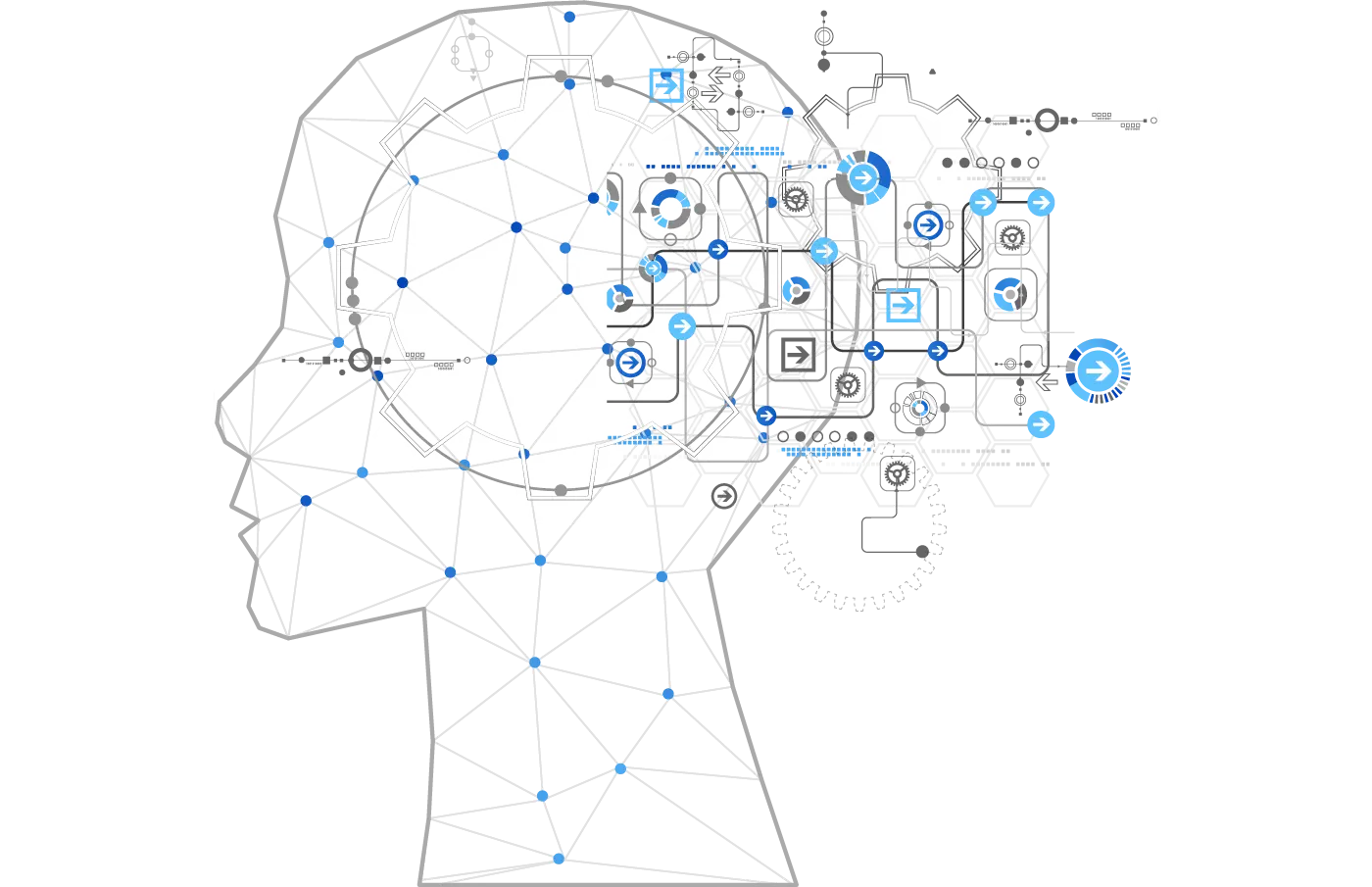
Information at a glance
- Conference Date: Thursday, September 3, 2020
- Conference Location: online (see Schedule for detailed links)
- Conference Topics: Artificial Intelligence in Industry and Finance
- Conference Schedule with Zoom links
- Conference Flyer(PDF 325,4 KB)
- Conference Program
- Program booklet(PDF 1,3 MB)
- Related Journal: "Frontiers in Artificial Intelligence"(PDF 266,5 KB)
Aim of the conference
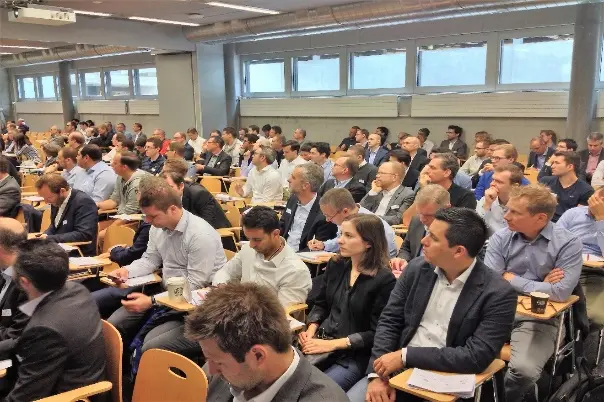
The aim of this conference is to bring together European academics, young researchers, students and industrial practitioners to discuss the application of Artificial Intelligence in Industry and Finance.
The 1st COST Conference was held on September 15, 2016, the 2nd COST Conference was held on September 7, 2017, the 3rd COST Conference was held on September 6, 2018, and the 4th AI Conference was held on September 5, 2019.
All lectures are open to the public.
Related Conferences
- Smart Maintenance Conference 2020 (September 8, 2020, Zürich)
- 4. Konferenz Perspektiven mit Industrie 4.0 (September 2, 2020, Winterthur)
- ANNPR 2020 (September 2-4, Winterthur)
Thematic Sessions
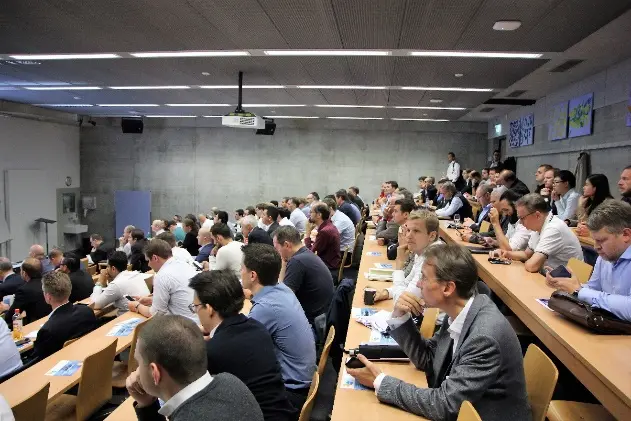
- Artificial Intelligence in Finance: Artificial Intelligence and Fintech challenges for the European banking and insurance industry
- Artificial Intelligence in Industry: Artificial Intelligence challenges for European companies from the mechanical and electrical industry, but also life sciences.
- Regulatory Technology in Finance: This session is devoted to the use of modern technology for the automation of regulatory reporting in finance.
- Ethical Questions in Artificial Intelligence: Issues arising in AI applications such as trust, explainability, neutrality, responsibility, moral consequences of algorithmic decisions.
Keynote Speaker
Martin Ulbrich, DG Connect (European Commission): "How to make Artificial Intelligence Ethical"
Invited Speakers
Artificial Intelligence in Finance
- Prof. Dr. Monica Billio, Università Ca' Foscari Venezia: "Bayesian Dynamic Tensor Regression for multilayer financial networks"
- Dr. Paul Bilokon, The Thalesians / Toby Weston, Imperial College: "Optimal Execution using Reinforcement Learning"
- Prof. Dr. Igor Halperin, Fidelity Investments: "Inverse Reinforcement Learning: applications in Finance"
- Prof. Dr. Wolfgang Härdle, Humboldt-Universität Berlin: "FRM the AI based Financial Risk Meter"
- Prof. Dr. Petter Kolm, NYU: "Hedging an Options Book with Reinforcement Learning"
- Dr. Kay Pilz, Kinetic Minds: "Machine Learning for Data Generation and Hedging in Finance"
Artificial Intelligence in Industry
- Jens Frühling, Accenture: "Bot and claims handling automation + automated damage assessment with AI"
- Dr. Helmut Hauser, University of Bristol: "The Body as a Computer – From Computing Octopus Arms to Sensing Spider Webs to Growing Robots"
- Daan Kakebeeke, Bain & Company: "Reinventing the Industrial base"
- Evelyn Menzel, Mindfire AG: "On the quest for human level AI through virtual reality"
- Prof. Dr. Thrishantha Nanayakkara, Imperial College: "Shared computation between the brain and the body"
- Dr. Liudmila Zavolokina, Ergon Informatik/Universität Zürich: "Blockchain for business"
Special Session "Regulatory Technology in Finance"
- Jefferson Braswell, Tahoe Blue Ltd: "Opportunities for the Innovative Application of Analytics in Financial Risk Management and Reporting"
- Angus Moir, Bank of England: "Digital Regulatory Reporting, automating the reporting process"
- Prof. Dr. Tim Weingärtner, HSLU: "Is DeReg the answer to DeFi? Impact of Blockchain on RegTech"
- Panel discussion "Regulatory Technology – Today’s Challenges and Opportunities for a Stable Future" with: PJ Di Giammarino, JWG, London; Francis Gross, European Central Bank, Frankfurt; The Honorable Allan I. Mendelowitz, ACTUS Financial Research Foundation, Washington; Moderation: Prof. Dr. Wolfgang Breymann, ZHAW
Special session "Ethical Questions in Artificial Intelligence"
- Corinna Hertweck, Dr. Tim Räz, ZHAW: "Algorithmic Decision Making and Social Justice: On the morals of Predictive Modeling"
- Dr. Michael Stuart, Université de Genève, Dr. Markus Kneer, UZH: "Artificial Responsibility"
- Karin Tien, Value Design: "Implementing CDR Strategies – Ways of Managing Privacy Risk from a Law and Ethical perspective(PDF 4,4 MB)"
- Dr. Andreas Theodorou, Umeå University: "Contextualising AI Governance Guidelines"
Participants
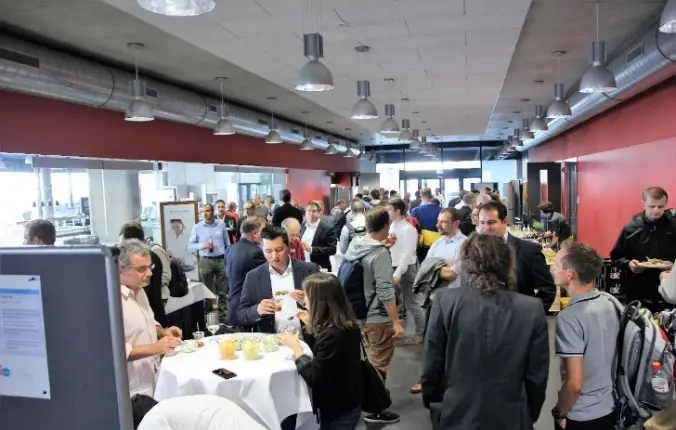
In 2017, 2018, and 2019, we have had around 200 participants, both from Academia and Industry. The latest instalment of the AI conference also saw a large number of international guests and speakers, travelling to Switzerland from destinations such as the UK, Germany, the United States and Bulgaria.
The largest proportion of participants will come from the industry complemented by a significant number of academic researchers. This mirrors our unique approach of connecting the academic world to their respective fields of application, putting new exciting concepts to work in industrial frameworks, where they can open up new opportunities.
Schedule
- Warm-up: 12:30-13:00
- Welcome: 13:00-13:10
- Keynote: 13:10-13:40
- Break: 13:40-14:00
- Thematic Sessions Part 1: 14:00-15:00. AI in Finance, AI in Industry, RegTech, Ethics
- Break: 15:00-15:30
- Thematic Sessions Part 2: 15:30-16:30. AI in Finance, AI in Industry, RegTech, Ethics
- Break: 16:30-17:00
- Closing: 17:00-17:30
Detailed Schedule
12:30-13:00 | Warm-up | |||
13:00-13:10 | Intro: D. Wilhelm: "Welcome and Introduction" | |||
13:10-13:40 | Keynote: M. Ulbrich: "How to make Artificial Intelligence Ethical" | |||
13:40-14:00 | Break | |||
AI in Finance 1 | AI in Industry 1 | Reg. Tech. in Finance 1 | Ethical Questions 1 | |
14:00-14:20 | K. Pilz: "Machine Learning for Data Generation and Hedging in Finance" | T. Nanayakkara: "Shared computation between the brain and the body" | T. Weingärtner: "Is DeReg the answer to DeFi? Impact of Blockchain on RegTech" | K. Tien: "Implementing CDR Strategies – Ways of Managing Privacy Risk from a Law and Ethical perspective" |
14:20-14:40 | P. Bilokon / T. Weston: "Optimal Execution using Reinforcement Learning" | E. Menzel: "On the quest for human level AI through virtual reality" | A. Moir: "Digital Regulatory Reporting, automating the reporting process" | A. Theodorou: "Contextualising AI Governance Guidelines" |
14:40-15:00 | W. Härdle: "FRM the AI based Financial Risk Meter" | H. Hauser: "The Body as a Computer – From Computing Octopus Arms to Sensing Spider Webs to Growing Robots" | J. Braswell: "Opportunities for the Innovative Application of Analytics in Financial Risk Management and Reporting" | |
15:00-15:30 | Break | |||
AI in Finance 2 | AI in Industry 2 | Reg. Tech. in Finance 2 | Ethical Questions 2 | |
15:30-15:50 | I. Halperin: "Inverse Reinforcement Learning: applications in Finance!" | L. Zavolokina: "Blockchain for business" | Panel Discussion "Regulatory Technology – Today’s Challenges and Opportunities for a Stable Future" | C. Hertweck / T. Räz: "Algorithmic Decision Making and Social Justice: On the morals of Predictive Modeling" |
15:50-16:10 | P. Kolm: "Hedging an Options Book with Reinforcement Learning" | J. Frühling: "Bot and claims handling automation + automated damage assessment with AI" | (Cont.: Panel Discussion) | M. Stuart / M. Kneer: "Artificial Responsibility" |
16:10-16:30 | M. Billio: "Bayesian Dynamic Tensor Regression for multilayer financial networks" | D. Kakebeeke: "Reinventing the Industrial base" | (Cont.: Panel Discussion) | |
16:30-17:00 | Break | |||
17:00-17:30 | Closing: D. Wilhelm: Closing remarks | |||
Martin Ulbrich
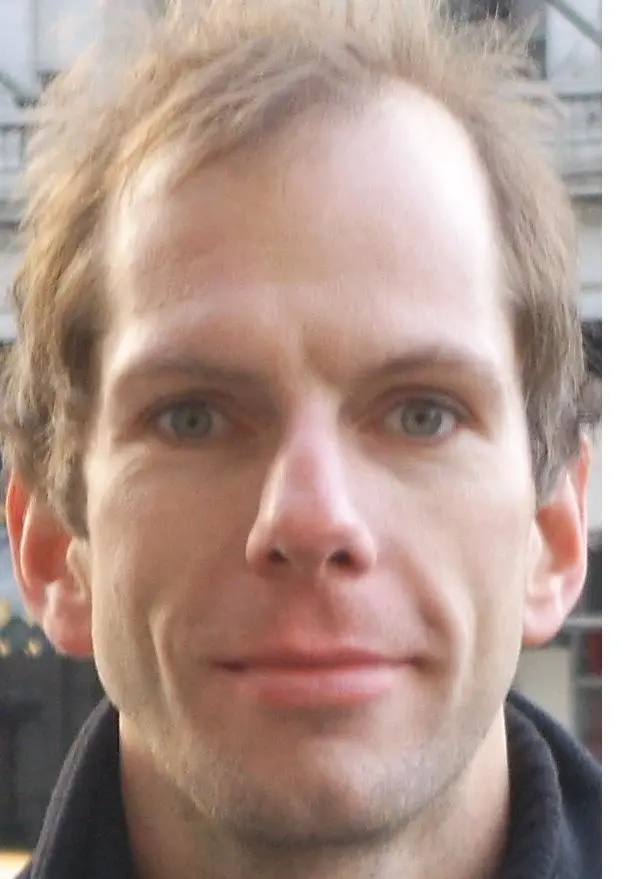
A Brief Biography
Martin Ulbrich, Senior Expert CNECT.A.2. An economist by training, Martin has been working on digital issues for more than twenty years in the Commission from different angles. Most recently, he has joined the AI policy team in 2018, contributing to the drafting of the White Paper on AI and working on the AI Regulatory Framework (He is part of the team that wrote the White Paper). Before joining the AI unit in 2018 he had worked most recently on the impact of digitisation on the labour market, and before that on geoblocking and on the economics of networks.
Mr. Ulbrich has previously worked among others in the European Commission’s Joint Research Center, where he analysed ICT research across the EU, as well as in its industrial policy and transport departments.
How to make Artificial Intelligence Ethical
He will present the Commissions policy approach in the follow-up to the White Paper on AI.
Prof. Dr. Monica Billio
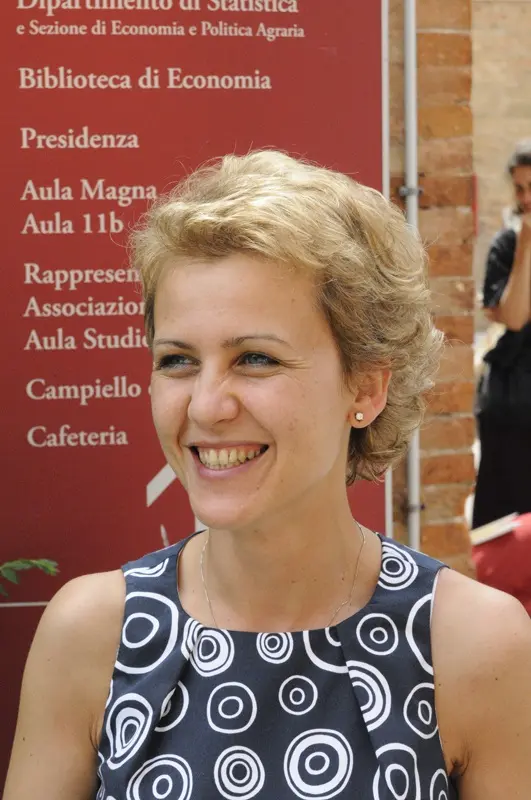
A Brief Biography
Full Professor of Econometrics at the University Ca’ Foscari of Venice. PhD in Applied Mathematics at the University Paris Dauphine.
Her main research field is Econometrics and its financial and economic applications. Her contribution are both methodological and applied. She contributed to the literature on simulation based methods and also on the Bayesian field. She devoted a lot of work to Markov switching models, pioneering their application in Finance and she continues to be a reference author for this type of models, in particular in the presence of latent components, which requires the use of simulation based techniques. She contributed to multivariate GARCH modelling, also integrating Markov switching components. In the last 10 years, she has devoted her research to analysing financial crises and systemic risk. Prof. Billio’s work on the field is nowadays very well recognized and she is often invited as an expert in conferences and round tables. The joint paper with Getmansky, Lo and Pelizzon (JFE 2012) is considered one of the main reference papers on the analysis of systemic risk and it draws the attention of academics, practitioners and regulators. In this paper, the idea of interconnectedness is introduced along with a relevant network structure. Starting from this initial work on networks, she built several lines of research to i) improve the network extraction; 2) use network topology for signalling and more recently 3) integrate the time dimension in order to develop dynamic network models. Moreover, in the last two years, she added the climate change dimension to her research agenda, being nowadays one of the main driver of financial stability.
Prof. Billio has published more than 100 technical papers in refereed journals, handbooks, and conference proceedings in the areas of econometrics and financial econometrics, with applications to risk measurement, volatility modelling, financial crisis and systemic risk. She is participating to many research projects financed by the European Commission, European Investment Bank, Eurostat and the Italian Ministry of Research (MIUR). She has been scientific coordinator of the SYRTO project, EU-FP7 project devoted to systemic risk measurement and she is local coordinator of three H2020-EE-CSA project on Energy Efficiency (EeMAP, EeDaPP and EeMIPP). She is also the coordinator of the EIBURS project “ESG-Credit.eu” dealing with the integration of ESG factors and climate change in Credit Analysis and Rating. The results of these and other research projects have appeared in peer-refereed journals including Journal of Econometrics, Journal of Financial Economics, Journal of Applied Econometrics, Journal of Financial Econometrics, Journal of Banking and Finance and European Journal of Operational Research.
Prof. Billio is actively involved in the organization of several scientific meetings and, in 2002 she co-established a new series of international workshops devoted to credit and financial risks (CREDIT), which has now reached the nineteenth edition (http://www.greta.it/credit/credit.htm). She is regularly on the program committees of the major international conferences and workshops of her fields and is currently member of the Board of Directors of the European Financial Management Association (EFMA) and member of the Scientific Committee of the Italian Association Financial Industry Risk Managers (AIFIRM).
Bayesian Dynamic Tensor Regression for multilayer financial networks
High dimension and multi-array data are becoming increasingly available in biology, physics, neuroimaging and economics. Examples include multi-layer economic and financial networks, multidimensional panels and brain images. These multidimensional data have a suitable representation as tensors and this calls for appropriate econometric tools which prevent data reshaping and are directly interpretable.
We propose a new dynamic linear model for tensor-valued response variables and covariates, called the tensor autoregressive model (ART), that encompasses some well known econometric models as special cases. Then, we derive on orthogonalized impulse response function, which allows for studying shock propagation within and between each dimension of the tensor-valued data.
We apply the ART model for analyzing the temporal evolution of multilayer networks of international trade and outstanding credit. The investigation is complemented by an impulse response analysis for studying the propagation of shocks across countries, over time and between layers. We found that, irrespective of its origin, any shock propagates between layers, but financial shocks are more persistent than those on international trade.
Dr. Paul Bilokon
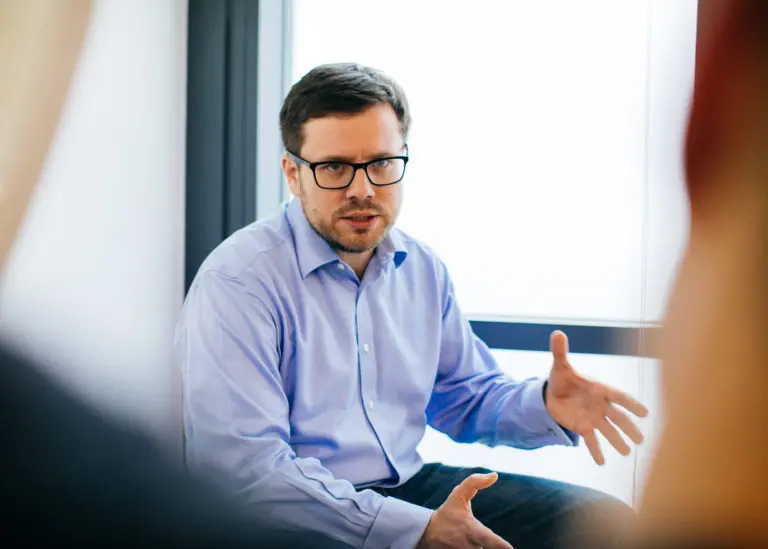
A Brief Biography
CEO and Founder of Thalesians Ltd. Previously served as Director and Head of global credit and core e-trading quants at Deutsche Bank, the teams that he helped set up with Jason Batt and Martin Zinkin. Having also worked at Morgan Stanley, Lehman Brothers, and Nomura, Paul pioneered electronic trading in credit with Rob Smith and William Osborn.
Paul has graduated from Christ Church, University of Oxford, with a distinction and Best Overall Performance prize. He has also graduated twice from Imperial College London.
Paul’s lectures at Imperial College London in machine learning for MSc students in mathematics and finance.
Paul has made contributions to mathematical logic, domain theory, and stochastic filtering theory, and, with Abbas Edalat, has published a prestigious LICS paper. Paul’s books are being published by Wiley, Springer, and World Scientific.
Toby Weston
Toby graduated with a Masters in Mathematics from Durham University, achieving distinction, in 2016. Since then he has spent two years working at JP Morgan Chase and is currently studying full time for an MSc in Mathematics and Finance at Imperial College London. His chosen thesis topic covers Reinforcement Learning for Optimal Execution.
Optimal Execution using Reinforcement Learning
Optimal execution involves finding a balance between the speed of execution and the minimisation of market impact. Prior work has shown that it has the potential to exploit idiosyncrasies of particular markets and beat basic benchmarks. Building upon this foundation we demonstrate the improvements that can be gained by applying recent developments in Reinforcement Learning to the trading environment.
Prof. Dr. Igor Halperin
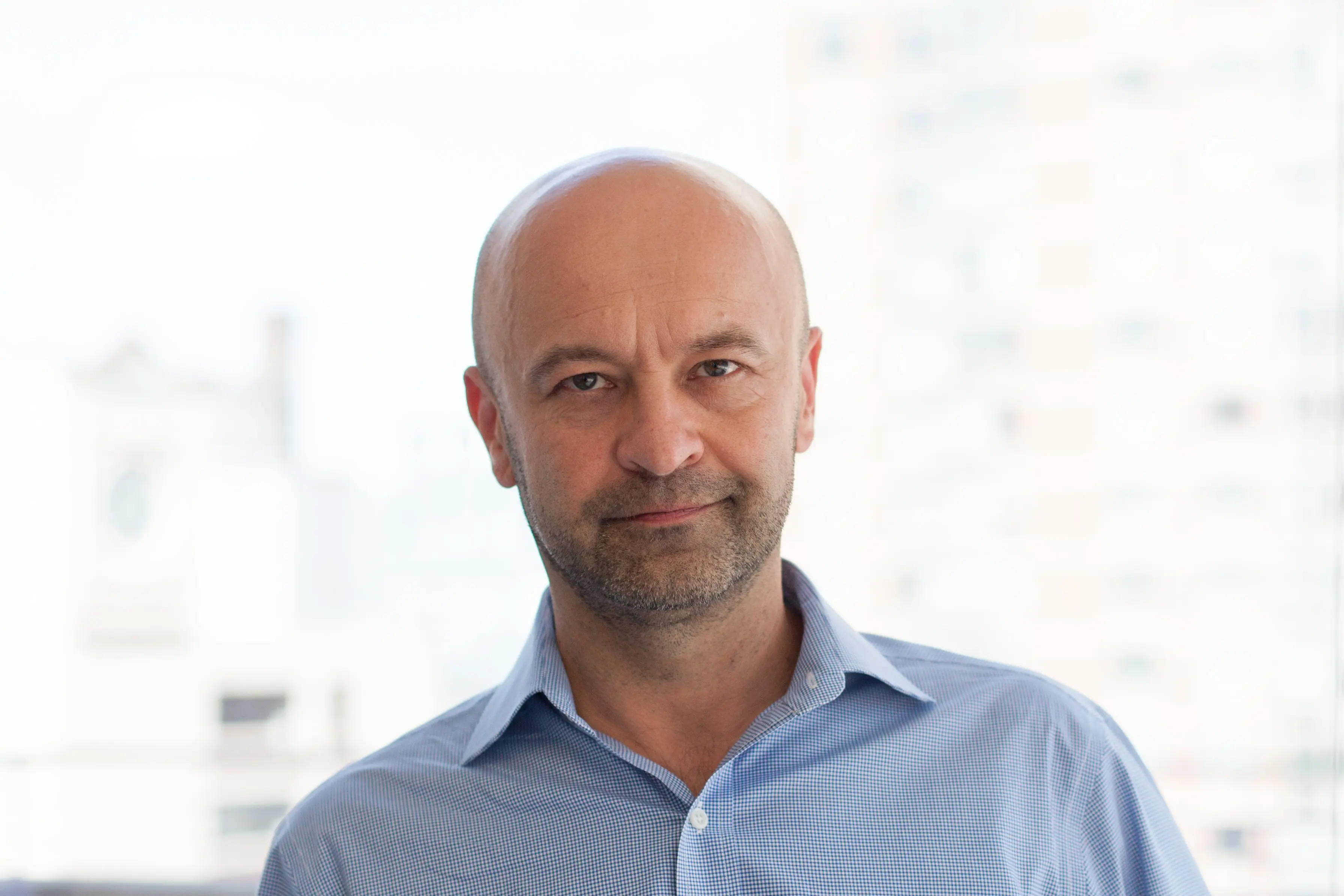
A Brief Biography
Igor Halperin is a researcher at Fidelity Investment and a Research Professor of Financial Machine Learning at NYU Tandon School of Engineering. His research focuses on using methods of reinforcement learning, information theory, neuroscience and physics for financial problems such as portfolio optimization, dynamic risk management, and inference of sequential decision-making processes of financial agents. Igor has an extensive industrial experience in statistical and financial modeling, in particular in the areas of option pricing, credit portfolio risk modeling, portfolio optimization, and operational risk modeling. Prior to joining Fidelity and NYU Tandon, Igor was an Executive Director of Quantitative Research at JPMorgan, and before that he worked as a quantitative researcher at Bloomberg LP. Igor has published numerous articles in finance and physics journals, and is a frequent speaker at financial conferences. He has also co-authored the books “Machine Learning in Finance: From Theory to Practice” (Springer 2020) and “Credit Risk Frontiers” (Bloomberg LP, 2012). Igor has a Ph.D. in theoretical high energy physics from Tel Aviv University, and a M.Sc. in nuclear physics from St. Petersburg State Technical University.
Inverse Reinforcement Learning: applications in Finance
In this talk, I will give a brief introduction to Inverse Reinforcement Learning (IRL), and give examples of its potential applications for quantitative finance.
Prof. Dr. Wolfgang Härdle
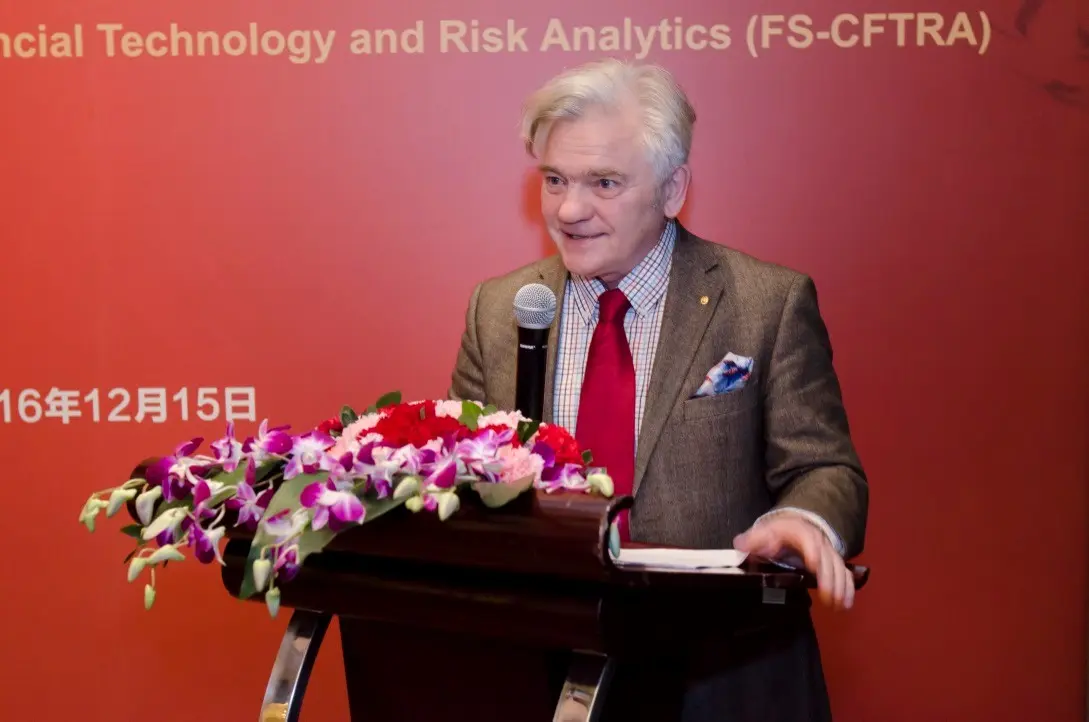
A Brief Biography
Wolfgang Karl HÄRDLE attained his Dr. rer. nat. in Mathematics at Universität Heidelberg in 1982 and in 1988 his habilitation at Universität Bonn. He is Ladislaus von Bortkiewicz Professor of Statistics at Humboldt-Universität zu Berlin and the director of the Sino German Graduate School (洪堡大学 + 厦门大学) IRTG1792 on “High dimensional non stationary time series analysis”. He also serves as head of the joint BRC Blockchain Research Center (with U Zürich). He is guest professor at WISE, Xiamen U, SMU, Singapore, NCTU, Hsinchu TW, Charles U, Prague CZ.
His research focuses on data sciences, dimension reduction and quantitative finance. He has published over 30 books and more than 300 papers in top statistical, econometrics and finance journals. He is highly ranked and cited on Google Scholar, REPEC and SSRN. He has professional experience in financial engineering, smart (specific, measurable, achievable, relevant, timely) data analytics, machine learning and cryptocurrency markets. He has created a financial risk meter, FRM hu.berlin/frm, a cryptocurrency index, CRIX thecrix.de. and organises regularly blockchainnights.com His web page is: hu.berlin/wkh
FRM the AI based Financial Risk Meter
(Andrija Mihoci, Michael Althof, Cathy Yi-Hsuan Chen, Wolfgang Karl Härdle)
A daily systemic risk measure is proposed accounting for links and mutual dependencies between financial institutions utilising tail event information. FRM (Financial Risk Meter) is based on Lasso quantile regression designed to capture tail event co-movements. The FRM focus lies on understanding active set data characteristics and the presentation of interdependencies in a network topology. Two FRM indices are presented, namely, FRM@Americas and FRM@Europe. The FRM indices detect systemic risk at selected areas and identifies risk factors. In practice, FRM is applied to the return time series of selected financial institutions and macroeconomic risk factors. Using FRM on a daily basis, we identify companies exhibiting extreme "co-stress", as well as "activators" of stress. With the SRM@EuroArea, we extend to the government bond asset class. FRM is a good predictor for recession probabilities, constituting the FRM-implied recession probabilities. Thereby, FRM indicates tail event behaviour in a network of financial risk factors.
Prof. Dr. Petter Kolm
A Brief Biography
Petter Kolm, Director of the Mathematics in Finance Master’s Program and Clinical Professor, Courant Institute of Mathematical Sciences, New York University
Petter Kolm is the Director of the Mathematics in Finance Master’s Program and Clinical Professor at the Courant Institute of Mathematical Sciences, New York University and the Principal of the Heimdall Group, LLC. Previously, Petter worked in the Quantitative Strategies Group at Goldman Sachs Asset Management where his responsibilities included researching and developing new quantitative investment strategies for the group's hedge fund. Petter has coauthored four books: Financial Modeling of the Equity Market: From CAPM to Cointegration (Wiley, 2006), Trends in Quantitative Finance (CFA Research Institute, 2006), Robust Portfolio Management and Optimization (Wiley, 2007), and Quantitative Equity Investing: Techniques and Strategies (Wiley, 2010). He holds a Ph.D. in Mathematics from Yale, an M.Phil. in Applied Mathematics from the Royal Institute of Technology, and an M.S. in Mathematics from ETH Zurich.
Petter is a member of the editorial boards of the International Journal of Portfolio Analysis and Management (IJPAM), Journal of Financial Data Science (JFDS), Journal of Investment Strategies (JoIS), Journal of Machine Learning in Finance (JMLF) and Journal of Portfolio Management (JPM). He is an Advisory Board Member of Betterment (one of the largest robo-advisors) and Alternative Data Group (ADG). Petter is also on the Board of Directors of the International Association for Quantitative Finance (IAQF) and Scientific Advisory Board Member of Artificial Intelligence Finance Institute (AIFI).
As a consultant and expert witness, Petter has provided his services in areas including alternative data, data science, econometrics, forecasting models, high frequency trading, machine learning, portfolio optimization w/ transaction costs and taxes, quantitative and systematic trading, risk management, robo-advisory and investing, smart beta strategies, transaction costs, and tax-aware investing.
Hedging an Options Book with Reinforcement Learning
In this talk we address the problem of how to optimally hedge an options book in a practical setting, where trading decisions are discrete and trading costs can be nonlinear and difficult to model.
Based on reinforcement learning (RL), a well-established machine learning technique we propose a model that is flexible, accurate and very promising for real-world applications. A key strength of the RL approach is that it does not make any assumptions about the form of trading cost. RL learns the minimum variance hedge subject to whatever transaction cost function one provides. All that it needs is a good simulator, in which transaction costs and options prices are simulated accurately.
Time permitting, we talk about a few different implementations of the RL algorithms (value, policy and DRL) and their impact on the hedging quality and how they generalize.
Dr. Kay Pilz
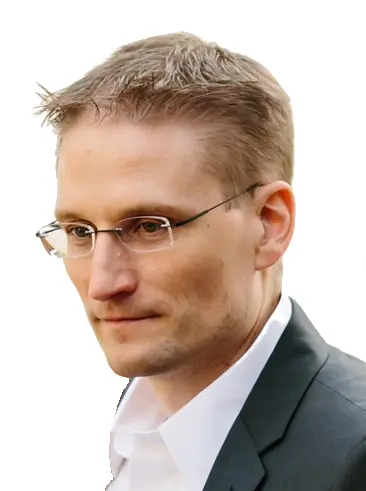
A Brief Biography
Kay is managing partner at kinetic mind GmbH and has a background in quantitative finance, statistical analysis and software development of more than 15 years.
Prior to his current position, Kay worked as a Senior Quantitative Analyst for STEAG, the fifth largest German energy provider, for E.ON Energy Trading, one of Europe’s largest energy provider, and for Sal. Oppenheim, an Investment Bank in Frankfurt, Germany. Kay developed and implemented pricing and hedging functionalities for exotic derivatives on equities, precious metals and energy commodities. He was also responsible for the development of productively used statistical prediction models employing methods from time series analysis and statistical learning.
Kay graduated in Mathematics from the University of Frankfurt and holds a PhD in Mathematical Statistics from the University of Bochum.
As a Senior Research Associate at the University of Technology in Sydney, Australia, he worked on a project on hybrid commodity and interest rate modelling, as well as on exotic option pricing in stochastic volatility models. Kay follows the latest research in the areas of quantitative finance and statistical learning, and publishes regularly in peer reviewed journals.
Machine Learning for Data Generation and Hedging in Finance
In this talk some applications of Machine Learning methods for data generation and deep hedging in the context of trading and risk management are presented. The first part discusses the use of generative neural networks for creating realistic market data like forward curves and volatility surfaces. The benefit of this data augmentation is demonstrated in the second part by fitting a deep hedger and evaluating its performance under realistic market conditions.
Prof. Dr. Thrishantha Nanayakkara
A Brief Biography
Dr. Thrishantha Nanayakkara is an associate professor in Design Engineering and Robotics at Dyson School of Design Engineering (DSDE), Imperial College London , where he is also the Director of the Morph Lab. He has published more than 140 papers in flagship robotics conferences and journals including IEEE transactions on robotics, IEEE Robotics and Automation Letters, RSS, IROS, ICRA, and RoboSoft. He is in the executive committee of the UK RAS Strategic Task Group for Soft Robotics, and in the editorial board as an Associate Editor of flagship robotics publications such as IEEE Robotics and Automation Letters, RSS, ICRA, IROS, RoboSoft, Frontiers in Soft Robotics, and the Journal of Robotics and Mechatronics. He has worked at leading laboratories for robotics and neuromotor control, including the Laboratory for Computational Motor Control, Johns Hopkins University, MIT Computer Science and Artificial Intelligence Lab (CSAIL), and Harvard Neuromotor Control Lab. He is and has been PI on EPSRC and EU funded projects of more than £5 million that have pushed the boundaries of our understanding on how conditioning the body improves the efficacy of action and perception in human-human and human-robot interactions.
Shared computation between the brain and the body
A system is called an embedded system if it can take good enough actions in response to states within deadlines imposed by the environment. In that sense living beings and most robots are embedded systems. When states are uncertain, the task of state estimation within deadlines becomes non-trivial. Living beings often take a recursive approach to estimate such random variables. For instance, if someone is asked to estimate the weight of an object, they would bob it up and down several times before concluding an estimate. If we frame it as a Recursive Bayesian estimation process, the agent can significantly benefit from the ability to “morph” the likelihood function to sharpen the posterior distribution. In our studies we see that participants change the elbow stiffness and bobbing behavior depending on the weight of the object in the above scenario. We see similar phenomena in other estimation tasks too. In soft tissue palpation for instance, when a Physician is required to estimate the location of the edge of the liver of a patient using manual palpation, they would regulate the stiffness and configuration of the fingers to condition haptic perception during palpation. In this talk, I will show some recent results of this information morphing approach for efficient estimation of environmental states using a controllable stiffness body. I will show a soft robotic approach to test hypotheses we build based on human behavior.
Evelyn Menzel
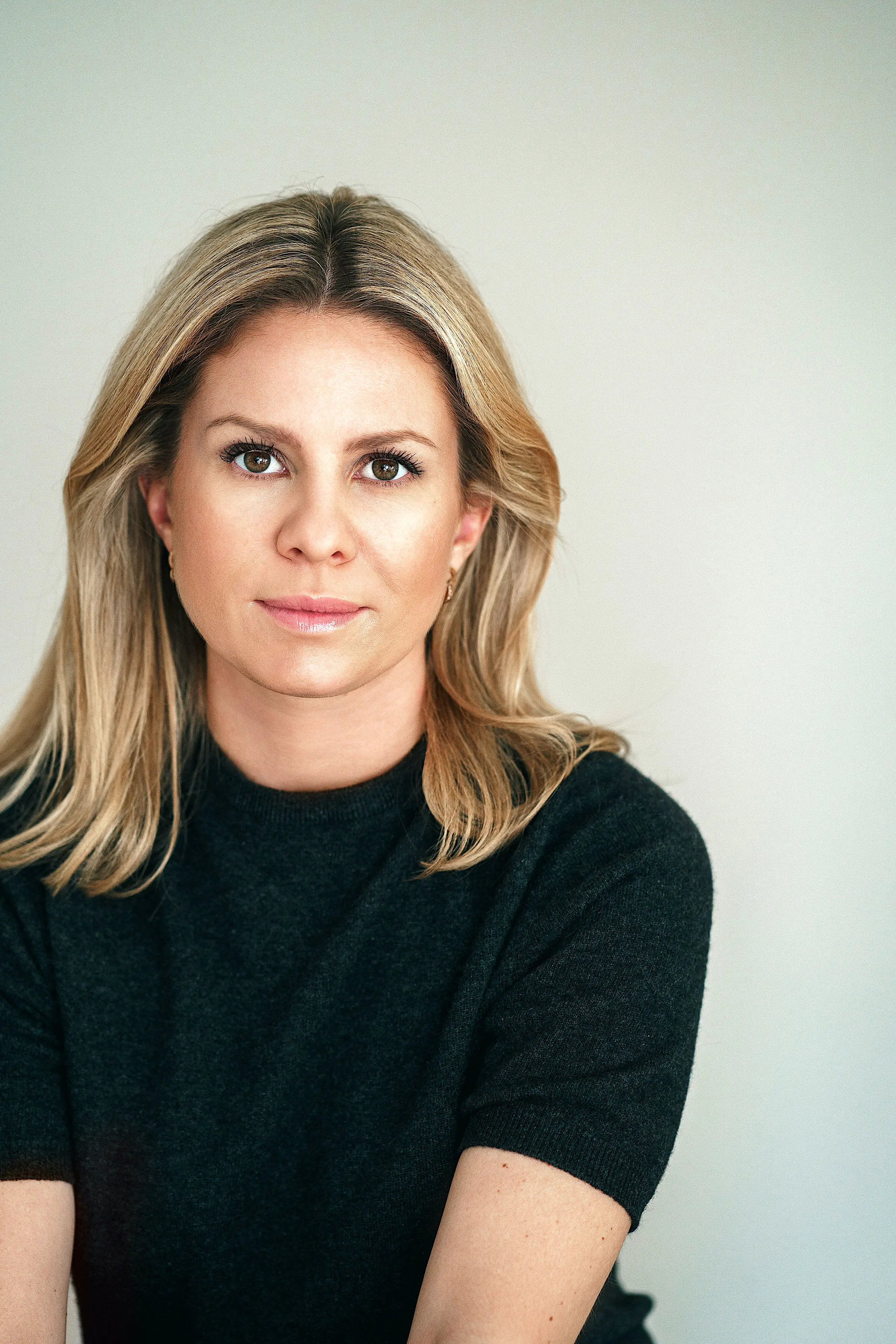
A Brief Biography
Evelyn is a tech entrepreneur and Chief Customer Officer for the AI startup Mindfire. Her mission at Mindfire is empowering clients and partners with technology, and shaping the world with AI to the better. She has worked over a decade with fortune 500’s in various technology and innovation driven projects, and is active in advising tech startups globally. Her background is economics, she lives in Zurich.
On the quest for human level AI through virtual reality
What tech companies call AI is often just a race for better automation or brute force computing.
To achieve human level Artificial Intelligence we need to be inspired by the renaissance geniuses, highly intelligent and transdisciplinary individuals. Mindfire‘s goal is to bring together some of the most creative individuals from different disciplines from around the globe in the world‘s largest virtual laboratory to create a new kind of collective superorganism or renaissance genius.
The development of new, innovative intelligent systems that drive the creation of human level AI requires a radical change in AI research direction.
Dr. Helmut Hauser
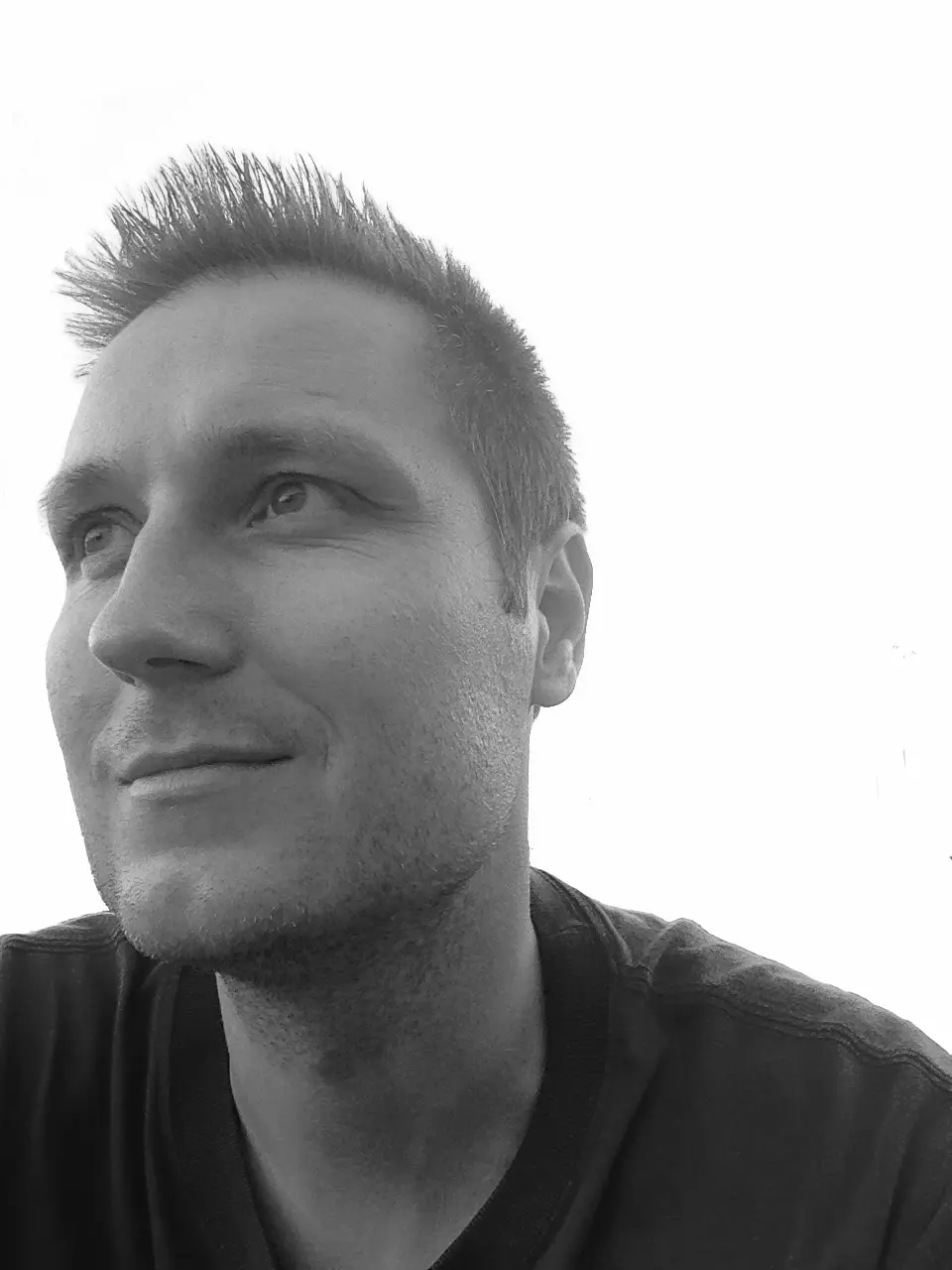
A Brief Biography
Helmut Hauser is a Senior Lecturer (Associate Professor) at the Department of Engineering Mathematics at the University of Bristol. He is a leading researcher in morphological computation and embodied intelligence. He seeks to understand the underlaying principles of how complex physical properties of biological systems are exploited to facilitate learning and controlling tasks, and how these principles can be employed to design better robots and novel sensor technologies. He has published over 60 papers including in high impact journals like Science Robotics, Nature Machine Intelligence and Scientific Reports. He won multiple awards including various “Best paper” awards and the “Highly Commended - Industrial Robot Journal award for practical innovation in the field of robotic”. He is currently leading the highly interdisciplinary Leverhulme Trust project “Computing with spiders’ webs". He is also the director of the EPSRC Centre of Doctoral Training in Future Autonomous and Robotic Systems and he is leading the UKRI Strategic Task Group on Soft Robotics.
The Body as a Computer – From Computing Octopus Arms to Sensing Spider Webs to Growing Robots
Despite the remarkable success of robotics, biological systems are still outperforming machines in almost any task. While robots are excellent at moving fast and precisely, they are surprisingly bad in robustness, adaptivity, energy efficiency and behavioral richness. It's speculated that one reason for this superiority of biological solutions is that nature exploits body dynamics for intelligent control, sensing, and to facilitate any underlying learning problems.
In our field of research, called morphological computation, we try do understand how nature is able to find embodied solutions through the evolutionary process, and how we can transfer this knowledge in better machine designs.
We will present three examples from our lab that show how bio-inspired machines can take advantage of this approach including a silicone-based octopus arm that can compute, a spider-web inspired vibration sensor that can classify signals, and growing robots that can adapt to their environments.
Dr. Liudmila Zavolokina
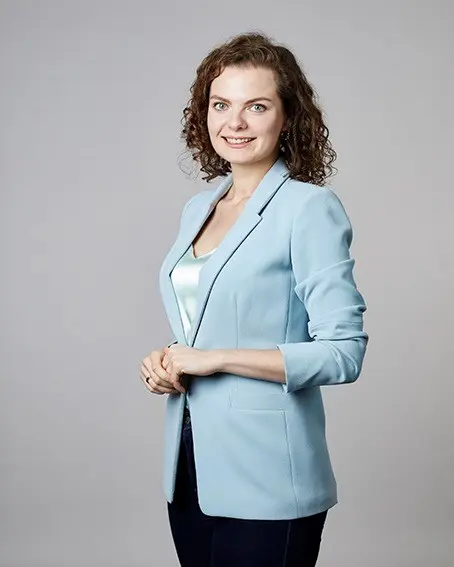
A Brief Biography
Liudmila Zavolokina is an IT consultant at Ergon Informatik and a postdoctoral researcher in Information Systems at the Information Management Research Group at the University of Zurich. She’s also a member of the Blockchain Center of the University of Zurich. Her research includes blockchain platforms and their impact on trust relationships, blockchain business models, and digital innovation in the financial area (FinTech). During her PhD, Liudmila co-initiated the Cardossier project – the Swiss blockchain ecosystem for car’s history - and led its research team. Today, Liudmila helps businesses leverage potential of blockchain technology and develop innovative solutions.
Blockchain for business
Blockchain has been around for a long time but, as the hype dies down, this security-based technology continues to challenge the business status quo across many organisations and industries. So, what does it look like behind the scenes of the technology everyone is talking about? In this talk, I show an approach that businesses may use to assess the usefulness of blockchain technology , and discuss potentials and benefits of blockchain technology for value creation.
Jens Frühling
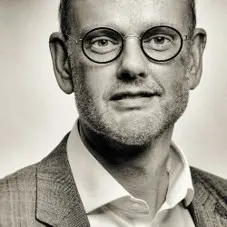
A Brief Biography
Jens Frühling is a Principal Director from Accenture, he joined Accenture in January 2017, having previously had leading positions in the areas of analytical Customer Relationship Management, Big / Smart Data and Digital Marketing in Banking. He benefits from 20 years of experience in management of complex BI and campaign automation projects.
Today he is responsible for GoTo Market of artificial intelligence (AI) Use Cases with the goal to establish Enterprise AI in all industries. Before that he built the artificial intelligence (AI) delivery capability within Accenture Applied Intelligence.
Jens holds a degree in Geography with specialization in geo-based marketing from Johann Wolfgang Goethe University, Frankfurt / Germany.
In the past twenty years he is Speaker at home and abroad and author of publications on analytical CRM and in recent times for Artificial Intelligence.
Bot and claims handling automation + automated damage assessment with AI
Global players like Ping An Insurance / China are leading the way: Consistent use of AI to reduce costs and increase customer satisfaction at the same time!
The vision: Capturing and checking the coverage of simple claims in a single customer contact - if possible automated until payment. Automatic real-time recognition of loss event, object, location, time, etc. from the description of the loss event. Collection of all relevant information for the cover check - if desired also with AI supported document check.
The result: Claims experts are relieved and can concentrate on complex cases, while increased customer satisfaction through "immediate decision" or already as complete as possible data collection in the first contact.
Dan Kakebeeke
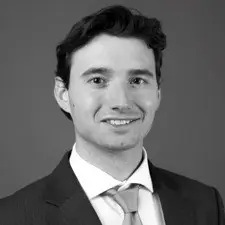
A Brief Biography
Daan Kakebeeke is a Senior Manager at Bain & Company. In the past he helped pioneer one of the earliest and most successful data science products for Bain in Financial Services. More recently he is a founding member of their Industrial Analytics team – which includes industry experts, operations consultants and data scientists. His work centers on effectively scaling digital technology and enterprise AI applications for industrial firms globally in sectors such as Energy, Chemicals and Manufacturing. As part of the work, he regularly interacts with leading vendors in the ecosystem. He holds a Bsc. in Chemistry and MBA degree at the university of Berkeley, with a 1-year specialization in Computer Science.
Reinventing the Industrial base
Industrial firms have been slow to adopt enterprise AI applications versus many other sectors and for good reason. It is hard to make AI work at scale in an industrial setting, in a way that adds value to operators and the business. However for those firms that get it right, the positive impact on operational performance and sustainability can be a true step-change. This talk will provide a brief history of industry developments and then will focus on some of the most promising Industrial applications of AI now and in the future as the industry starts to reinvent itself.
Angus Moir
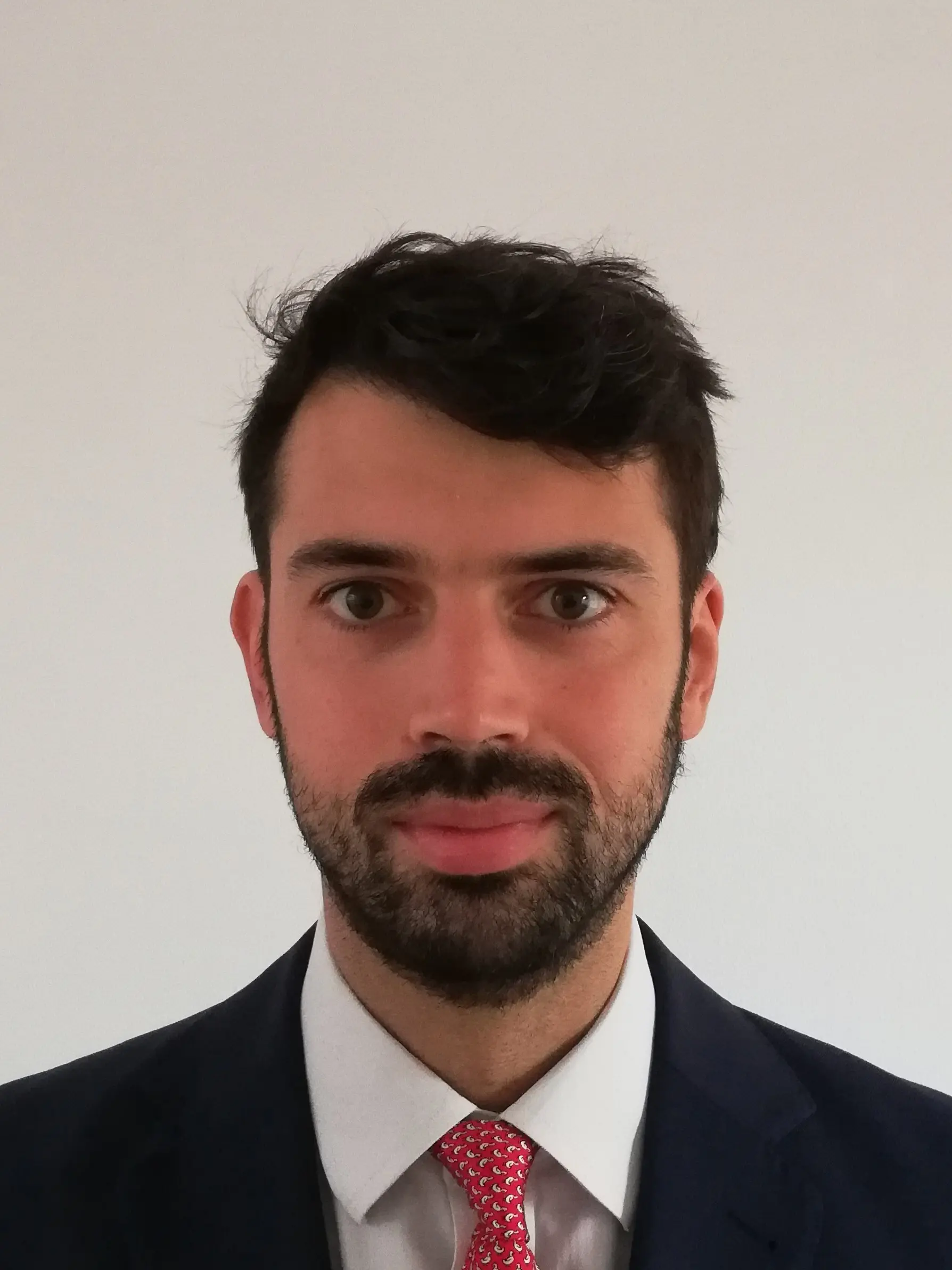
A Brief Biography
Angus Moir: Senior Manager, Data Collection Transformation team
Angus leads the data collection transformation team at the Bank of England: responsible for delivering a transformation of the way the Bank collects data from the UK financial sector. Previously, he led the Bank’s engagement in the Digital Regulatory Reporting initiative and played a key role in delivering a new supervisory dataset from the UK’s CCPs. Prior to dedicating his life to data and data collection, he held a number of roles at the Bank and in the private sector, primarily with a focus on risk analysis.
Originally an economist by training, his current primary interest, apart from improving data collection, is how to write rules and regulations in a “digital first” manner
Digital Regulatory Reporting, automating the reporting process
From 2016 to 2019, the Bank of England, FCA and participants from industry participated in a series of events under the banner of Digital Regulatory Reporting (DRR). At the events the UK financial regulators worked with industry to explore how the regulatory reporting process could be automated. DRR culminated with two six months pilots that developed a number of prototypes showcasing how automation may work in practice.
Prof. Dr. Tim Weingärtner
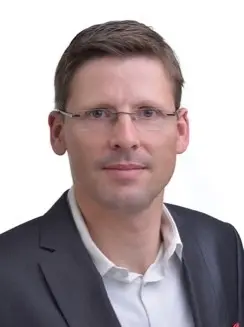
A Brief Biography
Prof. Dr. Tim Weingärtner is a lecturer at the School of Information Technology at the Lucerne University of Applied Sciences and Arts (HSLU), Switzerland. He is working on blockchain technology and its applications in IoT, identity and the energy sector. Furthermore he organizes the International Blockchain Forum Rotkreuz (ibfr.ch). As a representative in the Smart-up Program, he supports the promotion of young start-ups from the HSLU. As a member of the project team, he played a major role in setting up the Central Switzerland Innovation Park in Rotkreuz. Under the thematic focus "Building Excellence", the park deals with the Digital Transformation in the construction industry.
Before joining the university, Tim worked in the Swiss financial industry for more than 15 years. He led several major IT projects in credit management and credit risk management. During this time, he also worked as a product manager for the leading provider of core banking solutions in Switzerland. Tim studied computer science and received his doctorate in medical robotics from the University of Karlsruhe, Germany.
Is DeReg the answer to DeFi? Impact of Blockchain on RegTech
Decentralized Finance (DeFi) refers to financial applications developed on the basis of blockchain systems and currently focuses mainly on monetary banking services, peer-to-peer lending and tokenization. The regulation of blockchain based financial applications faces various challenges such as country-specific rules in globalized systems, high uncertainty or lacking technological knowledge. In this ecosystem, which is becoming increasingly complex on all fronts, new approaches are essential. The presentation will stimulate the discussion in this area and highlight some possible approaches.
Jefferson Braswell
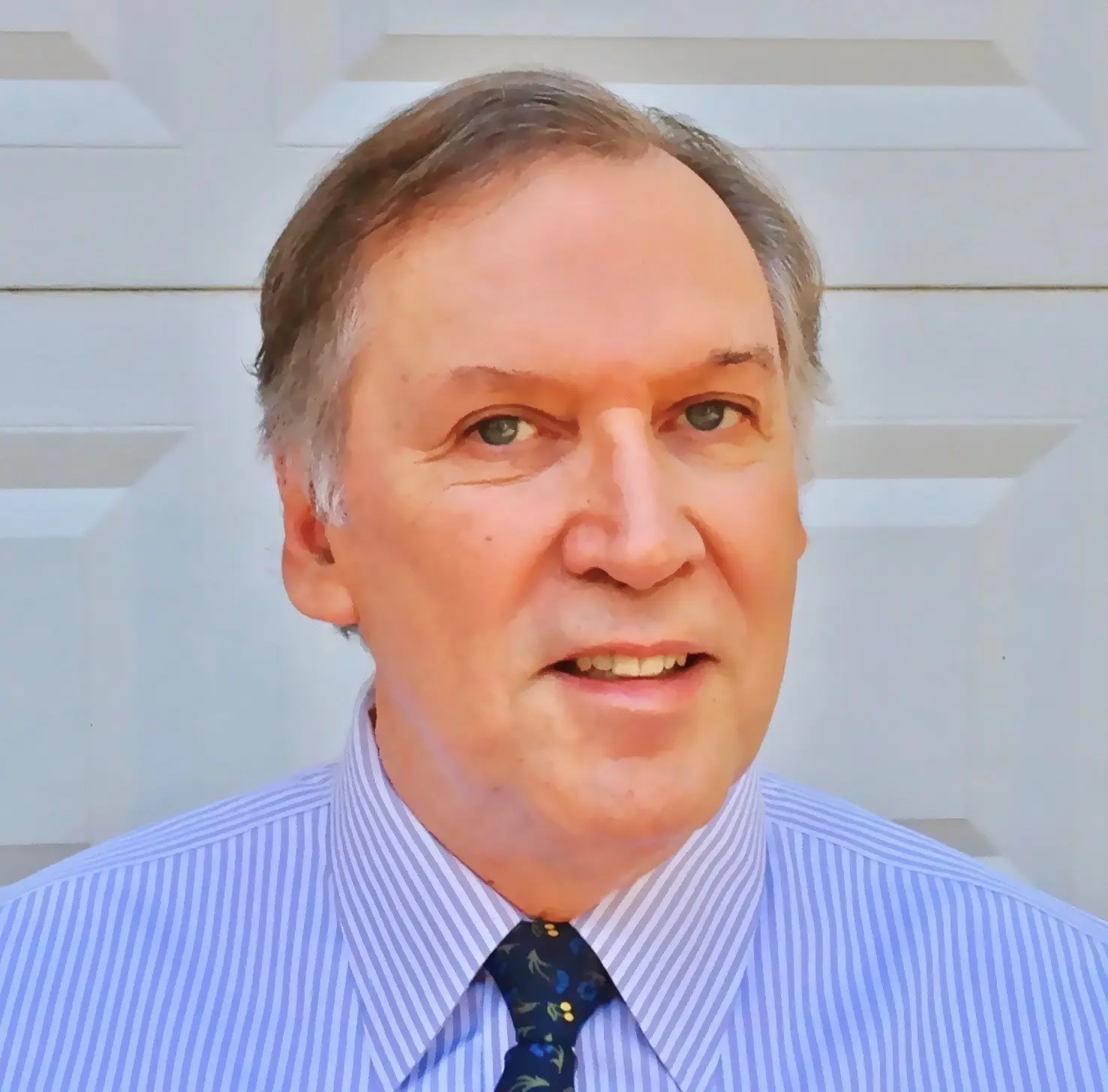
A Brief Biography
Jefferson Braswell has been successfully providing leading-edge business solutions for the financial sector for over 30 years. Tahoe Blue provides technology consulting, identification management services, and the development of enterprise risk models and data standards for the financial industry.
As one of its founding Directors, he has recently completed a 6-year term on the Board of the Global LEI Foundation (GLEIF), where he chaired the Technology, Operations and Standards Committee. He is also the Chair of the Board of Governors of the ACTUS Financial Research Foundation, and actively participates in several other ongoing financial data standards initiatives, including those of CPMI-IOSCO, ISO 20022 and ISO TC68 (Financial Services) and ISO TC307 (Blockchain) .
As co-founder and President of Berkeley-based Risk Management Technologies (RMT), Braswell designed and led the successful implementation of advanced, firm-wide risk management solutions integrated with enterprise-wide data management tools on high-performance computing platforms for many of the world's largest financial institutions, including Wells Fargo, Credit Suisse, Chase, PNC, Sumitomo Mitsui Banking Corporation, Mellon, Abbey National, Wachovia, Union Bank and ANZ.
Opportunities for the Innovative Application of Analytics in Financial Risk Management and Reporting
The availability of transparent -- and mathematically rigorous -- open-source standards for financial contract data and corresponding algorithms (such as ACTUS) has given rise to opportunities to leverage these standards as components in the application of innovative financial risk analytics in risk management applications. The opportunities to incorporate innovative analytics with a foundational platform that can generate cash flow projections from actual balance sheet contracts could be grouped, for convenience, into three basic stages: the input preparation stage, the cash-flow generation stage, and the output post-processing stage. The interaction of external risk models with internal contractual cash-flow algorithms in the generation stage is complex, and involves many factors. A careful delineation of the dynamics of such interactions is required in order to achieve the transparency, and explainable defensibility, of risk models. On the other hand, a discussion of opportunities to apply innovative analytical techniques in the input preparation stage and the output post-processing stage is more tractable, and this presentation will focus more on some of these opportunities.
Participants in the Panel Discussion "Regulatory Technology – Today’s Challenges and Opportunities for a Stable Future"
Panelists:
- PJ Di Giammarino, JWG, London
- Francis Gross, European Central Bank, Frankfurt
- The Honorable Allan I. Mendelowitz, ACTUS Financial Research Foundation, Washington
Moderator: Prof. Dr. Wolfgang Breymann, ZHAW
PJ Di Giammarino is an independent RegTech authority with a global network of senior bankers, regulators, and technologists which he brings together to enable compliance via adoption of new technology resulting in better, faster, cheaper and safer solutions.
Following a career of building systems and top management consulting including McKinsey, PJ was the COO of Technology at Barclays Capital.
Seeing the RegTech opportunity early, he founded JWG Group in 2006 to provide practitioners a platform for Joint Working Groups. As an independent think-tank JWG leverages its unique position with regulators, firms and their suppliers to facilitate the right RegTech dialogues and drive global change.
Currently JWG is working with the top players in the industry to deliver on the promise of digital regulatory reporting for global OTC derivatives and defining holistic management obligations for trade surveillance.
Since 2015, JWG has worked with global financial institutions to develop and deploy RegDelta - the gold standard for AI-powered control over regulatory obligations. Today we harness decades of intelligence to source tens of thousands of global regulatory texts and filter the noise to allow rapid risk assessment and effective control.
PJ has been based in Europe for 20 years and London for 15. He is an active member of the International Organisation for Standardization and he also serves as Chairman of the Committee to Establish the RegTech Council.
Francis Gross is Senior Adviser in the Directorate General Statistics of the European Central Bank.
Francis’ main interest lies in developing vision, sustainable conceptual design and strategy for overcoming the dual disruption of rapid globalization and digitization. The focus lies on measurement. The crisis taught us that we need to build measurement tools that will be effective at the scale and speed of finance also in 20-30 years, i.e. global and real time, especially in a crisis. The immediate aim is to design and drive the implementation of concrete, feasible measures with transformational power.
The underlying strategic credo is that to achieve that goal we must make the world more measurable. A simple first step is to build global data infrastructures that make global standards real for all. For that, authorities and the private sector must work together, globally, separating areas for cooperation from those for competition.
Francis’ immediate focus lies on the “real world - data world” interface, beginning with object identification, specifically legal entities. He serves on the Regulatory Oversight Committee of the G20-backed Global Legal Entity Identifier System (GLEIS) and has been instrumental from the start in the emergence and development of the GLEIS.
Prior to joining the ECB in 2001, Francis spent fifteen years in the automotive industry, eight of which at Mercedes, working mainly on globalisation, strategic alliances and business development.
He holds an engineering degree from École Centrale des Arts et Manufactures, Paris, and an MBA from Henley Management College, UK
In his free time, Francis coaches athletes for javelin throwing, including the current decathlon world champion.
The Honorable Allan I. Mendelowitz is President of the ACTUS Financial Research Foundation. The ACTUS Foundation is a not-for-profit corporation that is dedicated to creating and promoting an open-source fee-free algorithmic financial contract standard that enables current and forward-looking analysis. Over the course of his career he has held a number of senior executive government assignments. In his last position he served as Chairman of the Federal Housing Finance Board, the prudential regulator of the Federal Home Loan Bank system. He has published articles in scholarly journals and popular publications, lectured widely in the United States and abroad on economic and financial topics, and testified as an expert witness before the U.S. Congress more than 145 times. His education includes economics degrees from Columbia University (A.B.) and Northwestern University (Ph.D.).
Prof. Dr. Wolfgang Breymann is head of the group Finance, Risk Management and Econometrics at Zurich University of Applied Sciences, Institute of Data Analysis and Process Design, which he shaped by developing the research activities in financial markets and risk. After a career in theoretical physics, he turned to finance in 1996 as one of the early contributors to the then burgeoning field of Econophysics and joined ZHAW in 2004. He is one of the originators of project ACTUS for standardizing financial contract modelling and member of the board of directors of the ACTUS Financial Research Foundation as well as founding member of Ariadne Software AG and Ariadne Business Analytics AG. His current R&D interests are focused on the automation of risk assessment to improve the transparency and resilience of the financial system.
Wolfgang Breymann has managed large national and international projects. He authored or co-authored over 40 refereed papers and co-authored the book “Unified Financial Analysis”. He has given many invited talks at universities and conferences all over the world.
Karin Tien
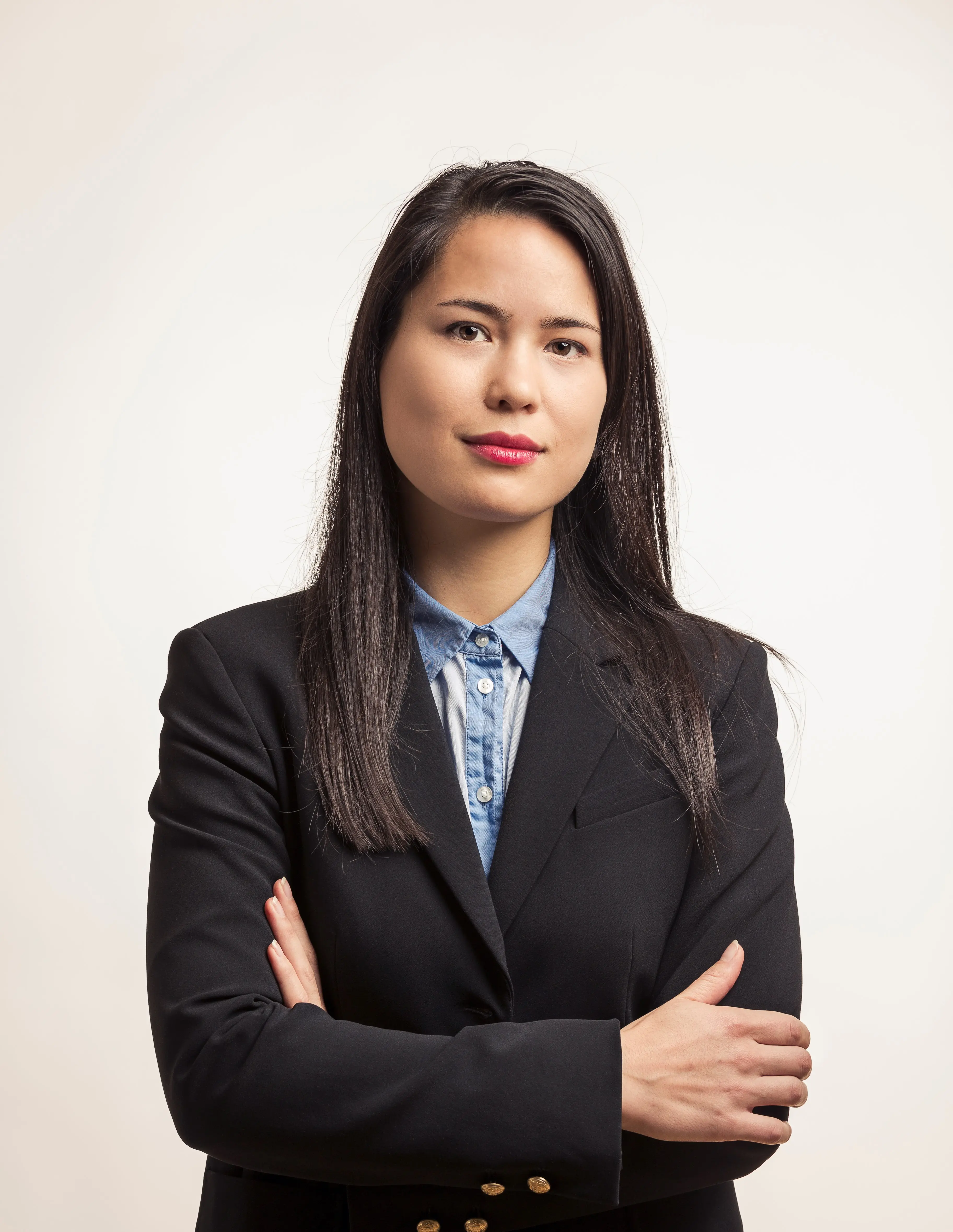
A Brief Biography
Karin Tien received her degree in Business Law in 2015 (University of Innsbruck, Tyrol, Austria) after she completed her education at the HTL Dornbirn in Chemical Engineering with focus on Textile Chemistry. Beside her diploma studies she worked from 2010 to 2015 in an IT-start-up, based in Vorarlberg, pioneering in the field of development of Mobile Apps which is why her master's degree thesis deals with legal requirements of “Bring your own device and mobile device management” (employee privacy issues).
After her judicial clerkship in the district of the Higher Regional Court Vienna she started as an associate in international law firms ingrained in the Technology, Media and Communications sectors. Her practice focused on providing legal advice on all matters relating to data protection law. Before founding a consultancy business, she passed the Austrian Bar Exam and worked at the Austrian Financial Market Authority (FMA) in areas of Crisis Management and Recovery Planning.
Over time, she mainly specialized in the field of data protection, both from the legal and from the organizational as well as from the management perspective. She performed implementations, assisted clients as data protection officer and external auditor. Furthermore, she creates Corporate Digital Responsibility (CDR) programs and ethical guidelines dealing with risks and privacy conflicts (trust vs. convenience of digital).
She authored or co-authored several papers about data protection as well as CDR and has given many invited talks at universities and conferences. Additionally, she supervises Master thesis (FHW Vienna) and professionally supports the Data Protection Think Tank at the Institute of Internal Auditors (IIA) in Austria.
Implementing CDR Strategies – Ways of Managing Privacy Risk from a Law and Ethical perspective
In many cases, prior to considering which ethics regulations AI Programs should comply with, it is often a beneficial approach to review the organization’s existing ethical standards. In various departments (eg HR, Sales, Marketing, R&D, Customer Service) different purposes for data use are given priority. This often leads to the fact that internal regulations based on a Corporate Digital Responsibility (CDR) program may help to harmonize the vision of values and to unite the actions of data processing.
For customers and conscious consumers, the existence of digital ethics programs is a sign of quality, as well as the basis of a long-term, trustful customer relationship. The experience of the past has shown that if there is no trust in the way an organization is processing data, the product or service might be rejected by the consumers and end users.
Today some organizations have already started to establish CDR programs. They have chosen different methods and strategies to address CDR and ethical issues in their development of software/products and data usage. This course of action is primarily driven by Corporate Social Responsibility (CSR) and Internal Audit Departments as they recognize risks (loss of reputation and customers, data breaches, non-acceptance of innovations) which could arise from blind spots.
This presentation will focus on different implementations of CDR strategies and gives a short overview about the key challenges organizations are facing in this context.
Dr. Andreas Theodorou
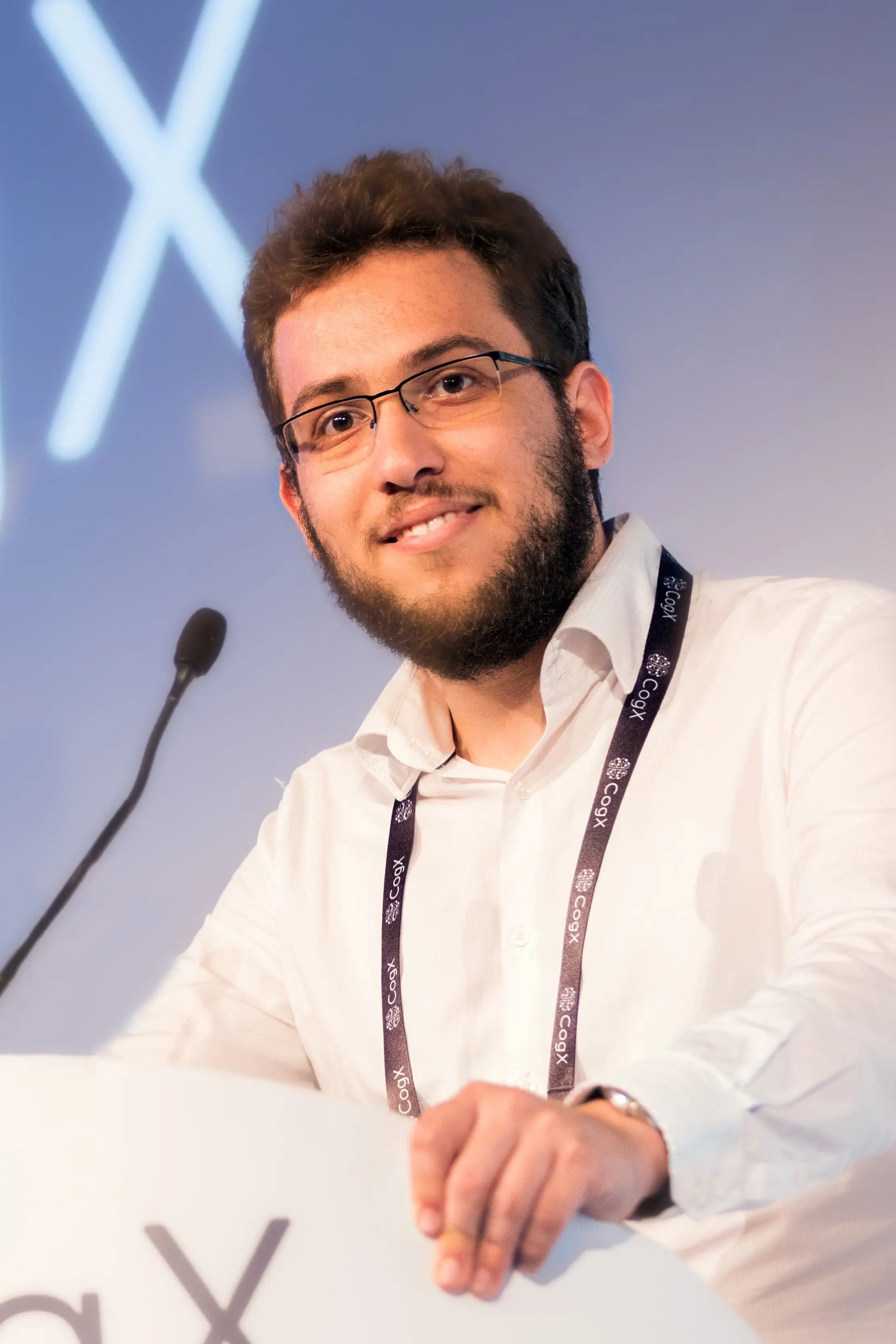
A Brief Biography
Dr. Andreas Theodorou is a postdoctoral researcher in the Responsible AI Group at Umeå University and the CEO and co-founder of VeRAI AB. His research interests include the development of software engineering methods of artificial intelligence, the verification and validation of ethical values in intelligent systems, the development means to provide transparency and explainability, and the study of the public’s perception of intelligent systems. In parallel to his research activities, Dr. Theodorou has been a contributing member of AI policy initiatives, e.g. IEEE SA’ P7001 series, ISO JTC1/42, UK’s AI APPG, EU’s AI Alliance, and others. He was part of the research team to evaluate the ethics guidelines for trustworthy AI suggested by the High-Level Expert Group on AI of European Commission. Dr. Theodorou has previously held research, visiting research, and teaching positions in the Georgia Institute of Technology (USA), University of Bath (UK), and University of Surrey (UK).
Contextualising AI Governance Guidelines
The last few years have seen a huge growth in the capabilities and applications of Artificial Intelligence (AI). Hardly a day goes by without news about technological advances and the societal impact of the use of AI. Not only are there large expectations of AI's potential to help to solve many current problems and to support the well-being of all, but also concerns are growing about the impact of AI on society and human wellbeing. Currently, many principles and guidelines have been proposed for “trustworthy” AI. They often rely on context-specific ethical socio-legal values — for example, fairness — but do not address the cultural variety between the different societies affected by AI system. Instead of more “one-size-fits-all” policies, in this talk I discuss socio-technical solutions to make such values explicit and, therefore, auditable.
Corinna Hertweck, Dr. Tim Räz
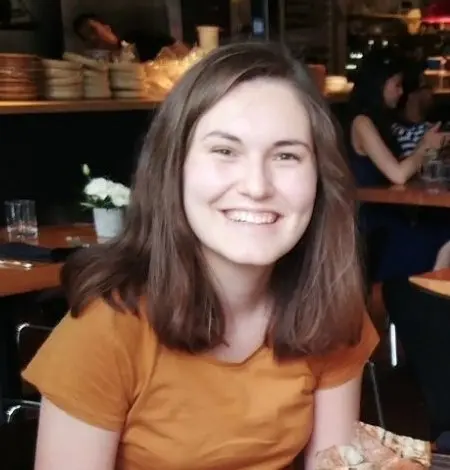
A Brief Biography
Corinna Hertweck is a PhD candidate at the Zurich University of Applied Sciences and the University of Zurich. Prior to starting the joint PhD program in 2020, she worked as a software engineer and graduated from the University of Helsinki with a master's degree in computer science. In her research, she focuses on the intersection of algorithms and social sciences. In particular, she is interested in questions of fairness and ethics arising when applying machine learning in the fields of education, recruitment, migration and criminal justice.
Tim Räz is a postdoctoral researcher in philosophy. He is working on the project “Socially acceptable AI and fairness trade-offs in predictive analytics in recruitment and education/training” (part of NRP 77 “Digital Transformation”). He is based at the Institute of Biomedical Ethics and History of Science (IBME), University of Zürich. His background is in philosophy (MSc., University of Bern, 2006), philosophy of science (PhD, University of Lausanne, 2013) and mathematics (MSc., University of Bern, 2019). His research interests include philosophy of science, philosophy of AI, and in particular philosophical issues of machine learning, including interpretability, explainability, and algorithmic fairness.
Algorithmic Decision Making and Social Justice: On the morals of Predictive Modeling
Algorithmic decision making based on predictive modeling is an intrinsic part of many AI solutions in finance and other services. The underlying algorithms are typically developed for optimizing business goals such as profit maximization or risk management, but the derived decisions have a concrete impact on the lives of humans. Basic societal values such as equality, freedom, or justice are on stake, and often threatened, as it turns out in the implemented practice. People are treated differently, as intended and needed for the business case. However, many recent examples show that algorithms may systematically discriminate against specific social groups. As a consequence, involved companies often face major reputation problems.
In our talk, we show why typical prediction-based decision systems nearly automatically generate issues of social fairness. We show how fairness of a decision system can be measured and managed, in order to avoid or minimize these issues, and we present an overview on fairness measures that have been established in practice. Finally, we comment on the ethical and moral significance of choosing to implement a specific form of fairness.
Dr. Michael Stuart, Dr. Markus Kneer
A Brief Biography
Michael T. Stuart is a research fellow at the Centre for Philosophy of Science at the University of Geneva, where he is principal investigator on a research project concerning Artificial Imagination, and the role of AI in science. His PhD is from the Institute for the History and Philosophy of Science and Technology at the University of Toronto. He has since been a postdoctoral or visiting fellow at the Universities of Pittsburgh, Cambridge, Bielefeld, and the London School of Economics. He recently completed a fellowship at the Digital Society Initiative at the University of Zurich, and will soon begin a fellowship at the Carl Friedrich von Weizsäcker Center for Foundational Research at the University of Tübingen. Mike is pursuing sociological cum philosophical research on the degree to which machines can be said to “believe,” “be aware of,” “know,” “understand,” and “imagine.” Establishing which of these epistemic states can properly be predicated of artificial agents is important for debates concerning our ability to praise and blame these agents for their “actions,” as well as for developing legal and moral frameworks for the attribution of (at least partial) responsibility when things go wrong (or right).
Markus Kneer (MA: Oxford University, PhD: Ecole Normale Supérieure, Paris) is the Principle Investigator of the Guilty Minds Lab (Centre for Ethics, University of Zurich) and a fellow at the Digital Society Initiative (UZH). After research positions at Pittsburgh University and Columbia University he joined the University of Zurich, where he works on theory of mind, ethics, and AI broadly conceived. Currently, he is particularly interested in biases in criminal trials on the one hand, and the psychological fundamentals of human-robot interaction on the other.
Artificial Responsibility
Tomas Hauer (2020) identifies two strands of work in the ethics of AI: one which creates and applies ethical rules for AIs, and another which asks whether AIs could behave ethically, in principle. To this second question, Kestutis Mosakas (forthcoming) has recently argued that artificial agents cannot behave ethically because AIs would need to be conscious to be ethical persons. Hauer claims that we should give up on this second question because the dominant methodology is a priori arguments and thought experiments about deep philosophical concepts (like consciousness and intentionality) that will not be resolved in time to assist in answering this question. We disagree, and see value in the efforts of some to make more piecemeal progress by considering less than fully autonomous entities as potentially partially responsible. We agree with Hauer that the way forward should not be through a priori arguments and unrealistic thought experiments, so we adopt an experimental method, testing the analogies drawn between AIs, animals, and corporations (e.g., by Laukyte 2020) with respect to moral responsibility and blameworthiness, and especially the capacity to have a "mens rea."
Hauer, Tomas. 2020. ‘Machine Ethics, Allostery and Philosophical Anti-Dualism: Will AI Ever Make Ethically Autonomous Decisions?’ Society, July. https://doi.org/10.1007/s12115-020-00506-2.
Laukyte, Migle. 2020. ‘The Intelligent Machine: A New Metaphor through Which to Understand Both Corporations and AI’. AI & Society, July. https://doi.org/10.1007/s00146-020-01018-7.
Mosakas, Kestutis. forthcoming. ‘On the Moral Status of Social Robots: Considering the Consciousness Criterion’. AI and Society, 1–15. https://doi.org/10.1007/s00146-020-01002-1.
Organizing Committee
- Prof. Dr. Jörg Osterrieder
- Prof. Dr. Dirk Wilhelm
- Prof. Dr. Rudolf Füchslin
- Dr. Andreas Henrici
- Prof. Dr. Peter Schwendner
- Prof. Dr. Wolfgang Breymann
Program Comittee
- Prof. Dr. Jörg Osterrieder
- Prof. Dr. Dirk Wilhelm
- Prof. Dr. Wolfgang Breymann
- Prof. Dr. Ruedi Füchslin
- Dr. Andreas Henrici
- Prof. Dr. Peter Schwendner
- Prof. Dr. Marc Wildi
- Prof. Dr. Christoph Heitz