Machine Perception and Cognition Group
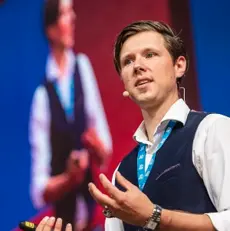
«KI ist DIE Schlüsseltechnologie des digitalen Wandels in allen Branchen und Sektoren und sie hat starke Auswirkungen auf unsere Gesellschaften. Darum leistet unsere Forschung wichtige Beiträge zu robusten und vertrauenswürdigen KI-Methoden, und wir vermitteln mit Begeisterung deren sichere Umsetzung und Anwendung.»
Expertise
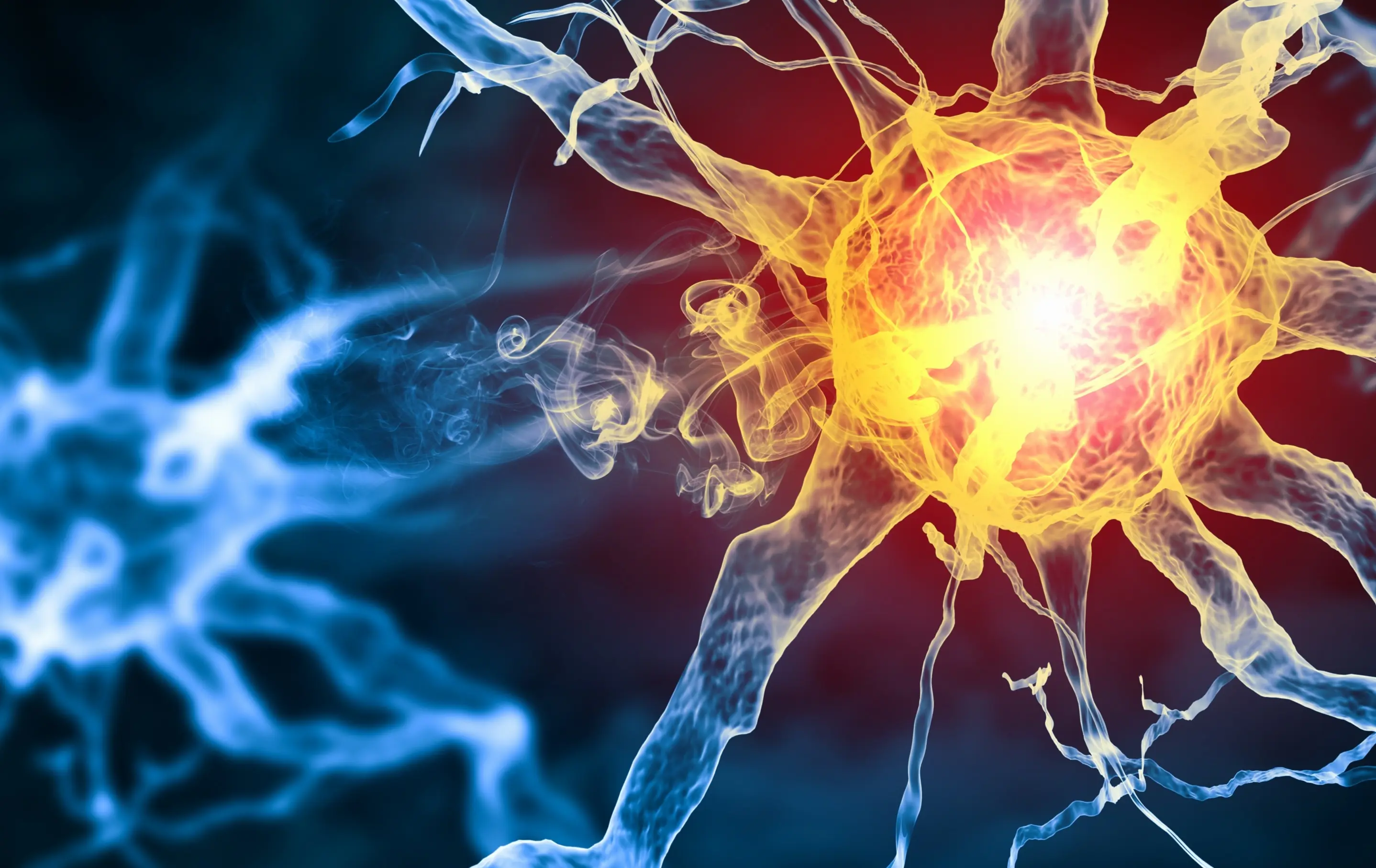
- Mustererkennung mit Deep Learning
- Maschinelle Wahrnehmung, Computer Vision und Sprechererkennung
- Entwicklung neuronaler Systeme
Die Machine Perception and Cognition-Gruppe forscht über Mustererkennung und arbeitet an einer Vielzahl von Aufgaben mit Bild-, Ton- oder allgemein Signaldaten. Wir befassen uns mit der Methodik der tiefen neuronalen Netze (Deep Neural Networks) und des Reinforcement Learning, inspiriert durch biologisches Lernen. Jede unserer Aufgaben hat ihr eigenes Lernziel (z. B. Erkennung, Klassifizierung, Clustering, Segmentierung, Novelty Detection, Steuerung) und ihren eigenen Anwendungsfall (z. B. vorausschauende Instandhaltung (Predictive Maintenance), Sprechererkennung für Multimedia-Indizierung, Dokumentanalyse, optische Notenerkennung, Computer Vision für industrielle Qualitätskontrolle, automatisiertes maschinelles Lernen, Deep Reinforcement Learning für automatisierte Spiele oder Gebäudeleittechnik). Diese werfen ihrerseits ein Licht auf verschiedene Aspekte des Lernprozesses. Wir nutzen diese Erfahrungen, um allgemeinere KI-Systeme zu kreieren, die auf neuronalen Architekturen basieren.
Angebote
- Einblick: Keynotes, Trainings
- KI-Beratung: Workshops, Expertenunterstützung, Beratung, Technikfolgenabschätzung
- Forschung und Entwicklung: kleine bis grosse Gemeinschaftsprojekte, Drittmittelforschung, studentische Projekte, praxiserprobte Prototypen
Team
Projekte
- Seite 01
- Seite 02
- Seite 03
- Seite 04
- Nächste Seite
-
Deep Dive ML on Simulated Enzyme-Electrolysis Performance
The goal of this pilot study is to research requirements needed to develop a computational model that simulates the fluidic and electro-biochemical dynamics in the power-to-liquid process in order to optimise the performance, efficiency and longevity of enzymes.
-
AI4REALNET – AI for REAL-world NETwork operation
The scope of AI4REALNET covers the perspective of AI-based solutions addressing critical systems (electricity, railway, and air traffic management) modelled by networks that can be simulated, and are traditionally operated by humans, and where AI systems complement and augment human abilities. It has two main ...
-
Stability of self-organizing net fragments as inductive bias for next-generation deep learning
We recently released "A Theory of Natural Intelligence", proposing a possible key to the emergence of intelligence in biological learners. Goal of this fellowship is to develop a technical implementation of the concept of self-organizing netfragments within contemporary deep artificial neural nets. ...
-
ML-BCA: Machine Learning für Body Composition Analysis
Das Centre for Artificial Intelligence (CAI) der ZHAW hat gemeinsam mit dem Kantonsspital Aarau im Rahmen von Vorstudien die Grundlage für Machine Learning-unterstützte Body Composition Analysis auf Bildbeständen des KSA gelegt und hierbei vielversprechende Ergebnisse erzielt. Ziel dieses Projekts ist die ...
-
Master3D – 3D-Master for a Digitized Manufacturing Platform
Wir erweitern die Real Time Manufacturing Services von Bossard durch die automatische Erstellung von Angeboten für Spezialteile. Das Herzstück ist ein von KI erstellter 3D-Master, der alle verfügbaren Teilinformationen vereint und die Preisfindung und Machbarkeitsbewertung für viele ...
-
certAInty – A Certification Scheme for AI systems
Certification of AI Systems by an accredited body increases trust, accelerates adoption and enables their use for safety-critical applications. We develop a Certification Scheme comprising specific requirements, criteria, measures, and technical methods for assessing Machine Learning enabled Systems. ...
-
DISTRAL: Industrial Process Monitoring for Injection Molding with Distributed Transfer Learning
We develop a distributed machine learning system to sort out defect plastic parts during production. Main challenge is the transferability of learnt process know-how from case to case; the solution builds on domain adaptation, continual data-centric deep learning and federated edge computing. ...
-
AC3T – AI powered CBCT for improved Combination Cancer Therapy
The project enables a novel, combined, adaptive cancer therapy combining tumor treating field and radiation therapy due to significantly improved static (3D) and time-resolved (4D) low dose Cone Beam Computer Tomography images based on artificial intelligence image reconstruction algorithms. ...
-
AUTODIDACT – Automated Video Data Annotation to Empower the ICU Cockpit Platform for Clinical Decision Support
Monitoring diverse sensor signals of patients in intensive care can be key to detect potentially fatal emergencies. But in order to perform the monitoring automatically, the monitoring system has to know what is currently happening to the patient: if the patient is for example currently being moved by medical staff, ...
-
Good practices for responsible development of AI-based applications in healthcare
This project will identify proven methods, practices and standards that support responsible research and development of AI systems for health. They will be tested in use cases from medical imaging and neurotechnology, publicly released and published as a guideline of recommended best practices. ...
Publikationen
-
Bolt, Peter; Ziebart, Volker; Jaeger, Christian; Schmid, Nicolas; Stadelmann, Thilo; Füchslin, Rudolf Marcel,
2024.
A simulation study on energy optimization in building control with reinforcement learning [Paper].
In:
11th IAPR TC 3 Workshop on Artificial Neural Networks for Pattern Recognition (ANNPR'24), Montreal, Canada, 10-12 October 2024.
ZHAW Zürcher Hochschule für Angewandte Wissenschaften.
Verfügbar unter: https://doi.org/10.21256/zhaw-31129
-
Jermain, Peter R; Oswald, Martin; Langdun, Tenzin; Wright, Santana; Khan, Ashraf; Stadelmann, Thilo; Abdulkadir, Ahmed; Yaroslavsky, Anna N.,
2024.
Deep learning-based cell segmentation for rapid optical cytopathology of thyroid cancer.
Scientific Reports.
14(1), S. 16389.
Verfügbar unter: https://doi.org/10.1038/s41598-024-64855-2
-
Dashti, Ali; Stadelmann, Thilo; Kohl, Thomas,
2024.
Machine learning for robust structural uncertainty quantification in fractured reservoirs.
Geothermics.
120(103012).
Verfügbar unter: https://doi.org/10.1016/j.geothermics.2024.103012
-
Yan, Peng; Abdulkadir, Ahmed; Aguzzi, Giulia; Schatte, Gerrit A.; Grewe, Benjamin F.; Stadelmann, Thilo,
2024.
In:
11th IEEE Swiss Conference on Data Science (SDS), Zurich, Switzerland, 30-31 May 2024.
ZHAW Zürcher Hochschule für Angewandte Wissenschaften.
Verfügbar unter: https://doi.org/10.21256/zhaw-30430
-
Meyer, Benjamin; Stadelmann, Thilo; Lüthi, Marcel,
2024.
ScalaGrad : a statically typed automatic differentiation library for safer data science [Paper].
In:
11th IEEE Swiss Conference on Data Science (SDS), Zurich, Switzerland, 30-31 May 2024.
ZHAW Zürcher Hochschule für Angewandte Wissenschaften.
Verfügbar unter: https://doi.org/10.21256/zhaw-30408
Sonstige Veröffentlichungen
Wann | Art | Titel |
---|---|---|
2023 | Extended Abstract | Thilo Stadelmann. KI als Chance für die angewandten Wissenschaften im Wettbewerb der Hochschulen. Workshop (“Atelier”) at the Bürgenstock-Konferenz der Schweizer Fachhochschulen und Pädagogischen Hochschulen 2023, Luzern, Schweiz, 20. Januar 2023 |
2022 | Extended Abstract | Christoph von der Malsburg, Benjamin F. Grewe, and Thilo Stadelmann. Making Sense of the Natural Environment. Proceedings of the KogWis 2022 - Understanding Minds Biannual Conference of the German Cognitive Science Society, Freiburg, Germany, September 5-7, 2022. |
2022 | Open Research Data | Felix M. Schmitt-Koopmann, Elaine M. Huang, Hans-Peter Hutter, Thilo Stadelmann, und Alireza Darvishy. FormulaNet: Ein Benchmark-Datensatz für die Erkennung mathematischer Formeln. Eine ungelöste Teilaufgabe der Dokumentenanalyse ist die Erkennung mathematischer Formeln (MFD). Forschungen von uns und anderen haben gezeigt, dass bestehende MFD-Datensätze mit Inline- und Display-Formel-Etiketten klein sind und eine unzureichende Etikettierungsqualität aufweisen. Es besteht daher ein dringender Bedarf an Datensätzen mit besserer Beschriftungsqualität für die zukünftige Forschung im Bereich MFD, da diese einen großen Einfluss auf die Leistung der darauf trainierten Modelle haben. Wir stellen eine fortschrittliche Etikettierungspipeline und einen neuen Datensatz namens FormulaNet vor. Mit über 45.000 Seiten ist FormulaNet unserer Meinung nach der größte MFD-Datensatz mit Inline-Formelbeschriftungen. Unser Datensatz soll bei der Bewältigung der MFD-Aufgabe helfen und kann die Entwicklung neuer Anwendungen ermöglichen, wie z. B. die Zugänglichkeit mathematischer Formeln in PDFs für sehbehinderte Benutzer von Bildschirmlesegeräten. |
2020 | Open Research Data | Lukas Tuggener, Yvan Putra Satyawan, Alexander Pacha, Jürgen Schmidhuber, and Thilo Stadelmann, DeepScoresV2. The DeepScoresV2 Dataset for Music Object Detection contains digitally rendered images of written sheet music, together with the corresponding ground truth to fit various types of machine learning models. A total of 151 Million different instances of music symbols, belonging to 135 different classes are annotated. The total Dataset contains 255,385 Images. For most researches, the dense version, containing 1714 of the most diverse and interesting images, is a good starting point. |